Using Statistics to Uncover the Truth About Individual Cells
Researchers at Penn have developed a better method for interpreting data from single-cell RNA sequencing technologies.
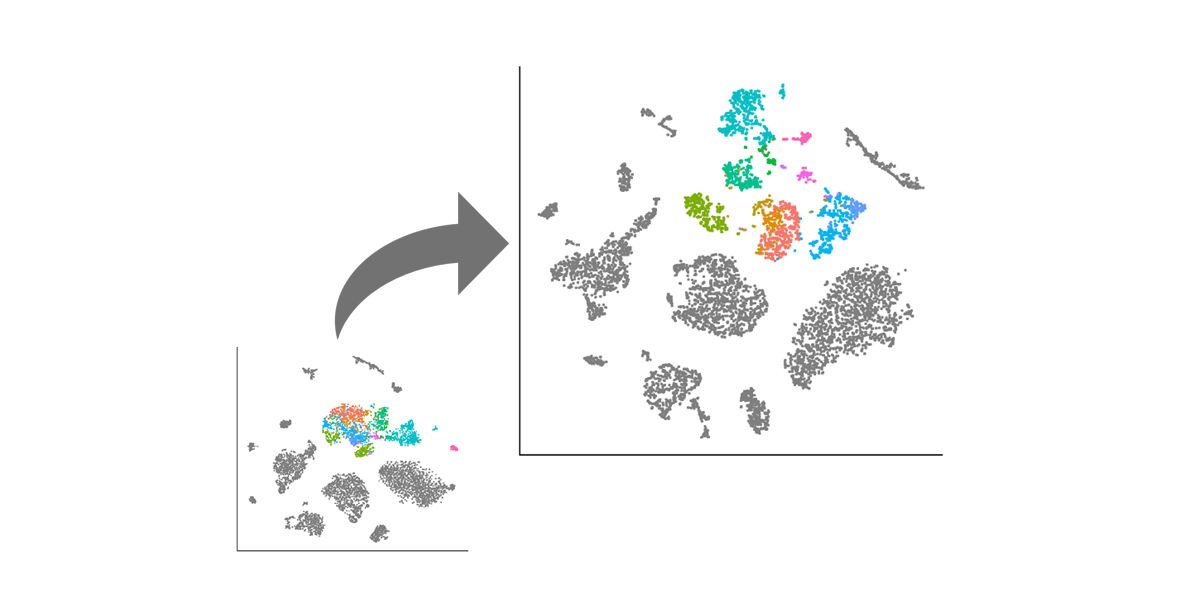
Until recently, genome-scale biology has been about looking at the average properties of cells all at once, which can make it difficult to learn about how individual cells differ from one another. But within the past five years, single-cell RNA sequencing technologies have matured, allowing researchers to measure the individual transcriptomes of many cells at once.
“Before single-cell sequencing came along, people did bulk sequencing [in which] you take a sample and measure the average gene expression across all of the cells,” says Nancy Zhang of the Statistics Department at the Wharton School. “You’re basically getting an average. That obscures the differences between cells in heterogeneous cell populations, which is critical for biological function.”
Single-cell sequencing opens doors for researchers to discover which cell types make up our body’s different tissues, such as in our eyes, brains, or livers, as well as how they behave and signal to each other — important steps in understanding human health and in diagnosing, monitoring, and treating disease.
But currently, the data produced by this kind of sequencing is extremely noisy and hard to interpret. Genes expressed at low levels often get lost in the data, and the observed gene expression distributions are, for most genes, distorted from the truth. These problems are becoming more severe as technologies that measure RNA abundances simultaneously for a large number of cells (thousands to millions) at extremely low depths become more common. In sequencing, “depth” is a concept akin to “sample size” in data collection: The higher the depth, the more accurate the characterization of each cell.
Researchers at Penn have developed two separate but complementary statistical techniques to analyze single-cell RNA sequencing data. The first, published in Nature Methods, de-noises the data, inferring what might have been seen in a much higher depth experiment. The other, published in the Proceedings of the National Academy of Sciences, is a “deconvolution method,” which infers the true gene-expression distribution across cells and examines how this distribution changes during development or disease.
The methods were developed by doctoral candidate Mo Huang and postdoctoral researcher Jingshu Wang, under Zhang’s guidance. This research is built on a collaboration between The Wharton School, Mingyao Li at the Perelman School of Medicine, and Arjun Raj’s group at the School of Engineering and Applied Science.
The de-noising method, called SAVER for “single-cell analysis via expression recovery,” imputes missing values and produces improved estimates of the expression level of each gene in each cell. Typically, with single-cell genomics, it is too costly to sample large numbers of cells and simultaneously sequence a large number of molecules from each cell. This results in sparse datasets with lots of missing data. SAVER amplifies the data, allowing researchers to get a bigger bang for their buck.
Compared to existing imputation methods, Zhang says SAVER is more cautious, only removing technical noise and preserving as much of the biological variation between cells as possible.
“In de-noising the data, it is tempting to be very aggressive. But one must avoid introducing artificial relationships between genes or artificial cell type patterns that are not real,” Zhang says. “That is a real danger because it is easy for spurious patterns to arise from pure noise in such high dimensional discrete data sets. Our goal in developing SAVER is to create a conservative, gentle method that does not over-smooth the data.”
The deconvolution method, called DESCEND for “deconvolution of single-cell expression distribution,” helps researchers estimate gene expression distributions across cells, allowing them to quantify aspects like the true biological variability of genes across cells or the proportion of cells where a gene is expressed. These are tricky to estimate because they are confounded by biological and technical factors, such as cell size or noise introduced by instruments. This new method allows researchers to more accurately characterize how each gene’s expression distribution changes across cells of different sizes, types, and conditions, leading to higher accuracy in selecting “important genes” for downstream analyses, such as clustering cells into distinct types.
For example, the researchers showed that the dispersion estimate produced by this method can be used to profile a population of cells going through differentiation. It turns out that genes with high dispersion in the early stages of differentiation are most important.
“If you apply DESCEND’s dispersion estimate to the early stages of differentiation,” Zhang says, “you are able to filter down to the important genes. If you just use the dispersion of the raw data without doing deconvolution, it turns out to be not trustworthy because it is influenced heavily by technical factors.”
One way the researchers assessed the accuracy of these methods, Zhang says, is by profiling the same population of cells using RNA Flourescence in situ hybridization (FISH), which is a more accurate but lower throughput technology. This crucial validation aspect of their research was contributed by the labs of Raj and John Murray of Medicine.
The raw single cell sequencing data look very different from the “gold standard” FISH data. But after de-noising by SAVER, or deconvolution by DESCEND, the sequencing and FISH data look very similar with matching distributions.
Single-cell sequencing is poised to transform the study of every branch of biology. One immediate impact is in the study of diseases. “In cancer, a tumor is made up of a bunch of cells that underwent mutations and transformed to become malignant,” Zhang says. “The problem is that these tumor cells…don’t all behave the same in response to treatment. One application of single-cell RNA sequencing is to sequence a tumor and see what cell populations there are, how they differ, and what genes they’re expressing to try to figure out how to best do therapy. That is already being done in labs around the world.”
Single-cell sequencing allows the cells and genes involved in disease to be identified, which is important both in understanding the fundamental biology and in diagnostics and treatment.
“You can imagine if this data comes from a diseased tissue and you want to know which genes, in which cell types, are driving the disease,” Zhang says, “you could look at the transformation from a normal cell population to a diseased population, or from a primary cancer population to a drug-resistant cancer population. This will allow you to identify new disease-relevant cell types that are key players in the process, and the genes that are driving the transition of cell to this type. Knowledge of target cells and target genes are key to developing better therapy.”
To follow up on this research, the developers of SAVER and DESCEND say they hope to apply these methods to a broad array of single-cell RNA sequencing studies to make more robust and reproducible analyses. The goal of this research, according to Zhang, is to give biologists the tools to squeeze the most out of their single-cell RNA sequencing data.
“Single-cell RNA sequencing has so much potential in the sense that, if this data were clean and you were able to trust it, people would be able to do so much with it,” she says. “The technology has been around for a few years but we’re still trying to grapple with what it can and cannot do. I’m hoping we can contribute by helping people get cleaner, better data and make meaningful inferences using these methods. Seeing signals pop out in the data is exciting.”
Nancy Zhang is a professor of statistics, Mo Huang is a doctoral candidate, and Jingshu Wang is a postdoctoral researcher, all in the Wharton School Statistics Department.