Penn Researchers Detect Brain Differences between Fast and Slow Learners
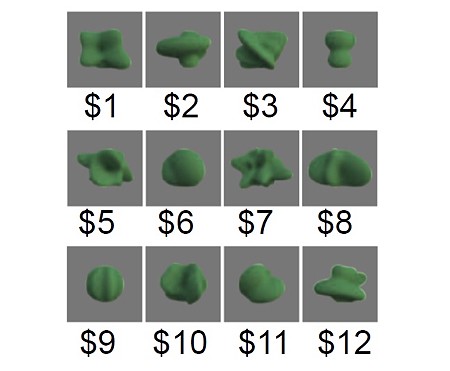
By Lauren Salig
Why do some people naturally excel at learning instruments, languages or technology while others take longer to pick up new knowledge? Learning requires the brain to encode information, changing its neural “wiring” and creating networks between brain regions.
In a new study, researchers at the University of Pennsylvania’s School of Engineering and Applied Science and the Max Planck Institute for Dynamics and Self-Organization in Germany looked at how brain activation patterns might affect how long it takes for new information to really stick in the brain.
Earlier research has suggested that part of what might slow down learners is over-thinking. A 2015 study led by Danielle Bassett, Eduardo D. Glandt Faculty Fellow and associate professor in the Department of Bioengineering, showed a correlation between slow learning and cognitive control: the brain’s ability to regulate itself by activating the necessary networks and inhibiting unnecessary activity. In that study, when people unnecessarily engaged parts of the brain linked to cognitive control, they were more likely to take longer to learn a simple task.
But beyond what might make an individual learn more slowly, the researchers want to know what sort of geometric patterns of brain activity make for better learning.
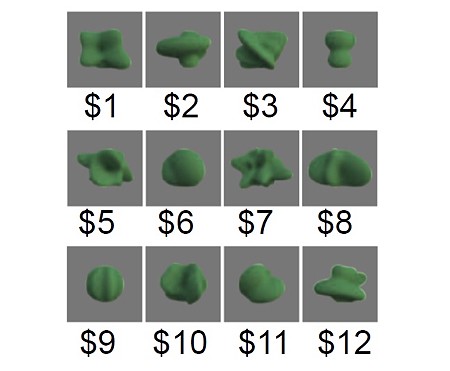
Their new study was led by Bassett and Evelyn Tang, who was an Africk Family Postdoctoral Fellow in Bassett’s Complex Systems Lab before starting at the Max Planck Institute this fall. Sharon Thompson-Schill, Christopher H. Browne Distinguished Professor and chair of Psychology, also contributed to the study.
The study was published in the journal Nature Neuroscience.
Tang, Bassett and their colleagues used fMRI scans to study the brains of 20 healthy individuals over the course of 4 days as they learned to associate geometric shapes with monetary values. For instance, participants might have learned that a mushroom-like shape had a value of $4 and a ribbed sphere had a value of $9. Then, they would need to use that learned information in tasks that required them to make value judgements based on the shapes. The task was developed by Marcelo G. Mattar, who was a graduate student with Bassett and Thompson-Schill before accepting a postdoctoral position, first at Princeton University and now at the University of Cambridge.
“All of our subjects learned the same information eventually, but some learned much faster,” Tang says. “We wanted to better understand the brain differences that might lead some to master the shape-number associations faster. Is activity in the brain separated in a way that is meaningful for the information being presented?”
To answer this question, the Penn researchers used a different approach than many other brain scientists by taking a page from machine learning. Instead of just identifying which brain areas were active, the researchers plotted geometric relationships within the brain, and then measured three main factors: embedding dimension, stimuli dimension and label assortativity.
To put these three factors into perspective, Tang uses a coffee shop analogy: “If you like Starbucks or you hate Starbucks, there should be a difference in the brain. But whether you love or hate Starbucks, your brain is going to ‘flash’ in either case. They would both be in the embedding dimension. It’s stimuli dimension that will show you the separation between the things you like and the things you hate. And label assortativity, like stimuli dimension, is measuring how easily things can be told apart.”
How did the brains of fast learners look different from those of slow learners in this study? Fast learners had higher stimuli dimension and label assortativity and lower embedding dimension on average, meaning their patterns of brain activity were easier to distinguish and more compact.
“The activity in their brain has a layout that is actually more information-containing than someone who learns less quickly,” Tang says. “For me, this is really cool because you can look at brain activity and see the different pattern.”
Participants’ ability to assign values to the novel shapes required them to use cognitive control, so the researchers are curious about how cognitive control might play into learning speed.
“Building compact, precise representations of these shape-value pairs requires the same sorts of complex cognitive processes that play a role in decision making, working memory and other so-called executive functions,” says Bassett.
“It would be interesting to explore if individuals with higher cognitive control or mental flexibility also learn faster, as the learning effectiveness we are seeing presumably depends on those qualities,” adds Tang.
Although the study’s insights into how the brain learns are an important accomplishment, Tang believes that a major contribution of their study is the methodology. Both label assortativity and stimuli dimension assess how the brain separates and stores information, but label assortativity is the more typical measurement in the field right now. The researchers wanted to introduce stimuli dimension as another valid measure that researchers can begin to use more.
“It isn’t bread and butter machine learning,” Tang says. “It uses machine learning in a way that gets more at dimension in space.”
By using a machine learning approach, the researchers were able to look at brain patterns more holistically, but they were also able to impartially verify past findings about specific brain areas involved in learning. The results indicated that the hippocampus and the right temporal pole were relevant for learning, consistent with other scientists’ findings.
“Crucially, we didn’t start out studying these areas, they just popped out of the analysis,” Tang says.
The study has important implications for brain science methodology moving forward, but Tang stresses that, in terms of real-world applications for improving learning, the study is only exploratory. At this point, the researchers are simply trying to understand how the brain learns.
“Down the road, people are thinking of things like intervening in the brain via technology, or by presenting the same material to the learner but in different ways,” Tang says. “If we can see that Pattern A is a better way of acquiring information than Pattern B, then maybe we can try to encourage Pattern A activity in the brain for more efficient learning.”
For now, those looking to learn a new instrument, language or skill will just have to make do with hoping to establish those efficient brain patterns on their own.
In addition to Evelyn Tang, Danielle Bassett and Sharon Thompson-Schill, the study was conducted by then-postdoctoral fellow at the Warren Center Chad Giusti, who is now an assistant professor at the University of Delaware, David Lydon-Staley, postdoctoral researcher in the Department of Bioengineering, and Marcelo Mattar.
The research was supported by the John D. and Catherine T. MacArthur Foundation, the Alfred P. Sloan Foundation, the ISI Foundation, the Paul Allen Foundation, the Army Research Laboratory (W911NF-10–2–0022), the Army Research Office (Bassett-W911NF-14–1–0679, Grafton-W911NF-16–1–0474, DCIST-W911NF-17–2–0181), the Office of Naval Research, the National Institute of Mental Health (2-R01-DC-009209–11, R01-MH112847, R01-MH107235, R21-M MH-106799), the National Institute of Child Health and Human Development (1R01HD086888–01), National Institute of Neurological Disorders and Stroke (R01 NS099348), and the National Science Foundation (BCS-1441502, BCS-101430087, NSF PHY-1554488 and BCS-1631550).