Paris Perdikaris’s Machine Learning Research on Nuclear Waste Featured by DOE
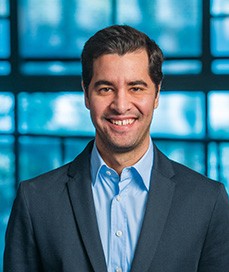
Paris Perdikaris, assistant professor in the Department of Mechanical Engineering and Applied Mechanics, was featured in the United States Department of Energy’s (DOE) ASCR Discovery for his research on using machine learning to predict nuclear waste leakage.
Perdikaris’s work, partially funded by his 2018 DOE Early Career Award, focuses on developing algorithms to approximate information about the physical world that is not directly accessible to scientists.
ASCR Discovery is published by the DOE’s Office of Advanced Scientific Computing Research and discusses how machine learning can be employed to find solutions to a diverse array of problems. The article details how Perdikaris implements machine learning to approach nuclear leakage at a decommissioned nuclear complex:
Quantifying the risks buried nuclear waste pose to soil and water near the Department of Energy’s (DOE’s) Hanford site in Washington state is not easy. Researchers can’t measure the earth’s permeability, a key factor in how far chemicals might travel, and mathematical models of how substances move underground are incomplete, says Paris Perdikaris of the University of Pennsylvania.
But where traditional experimental and computational tools fall short, artificial intelligence algorithms can help, building their own inferences based on patterns in the data. “We can’t directly measure the quantities we’re interested in,” he says. “But using this underlying mathematical structure, we can construct machine-learning algorithms that can predict what we care about.”
Continue reading at ASCR Discovery.