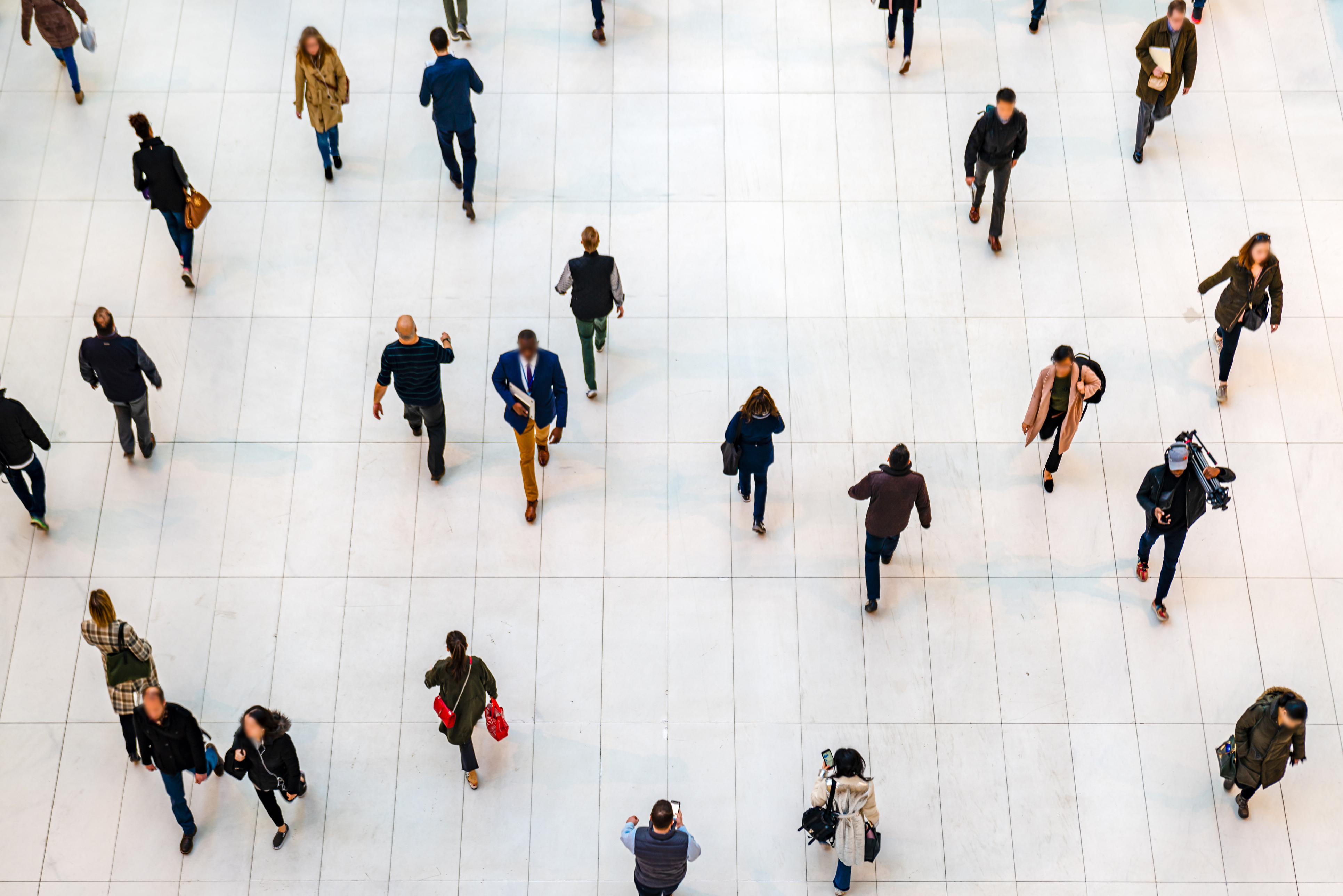
The year 2020 will go down in history as one drastically shaped by a virus that, as of late October, had infected more than 40 million people worldwide. Apt assessments have compared what’s happening now to the devastations of the 1918 flu pandemic. But what’s different today is how technology has allowed us to see, almost in real time, where the virus is spreading, how it’s mutating, and what effect it’s having on economies across the world.
This detailed view of COVID-19 is made possible thanks, in part, to a new generation of huge datasets—hundreds of genomes, millions of tweets—along with advances in computing power and the analytical methods to study them. Of course, massive datasets play different roles depending on the field using them. To provide some context, Penn Today spoke to experts across the University about how they and others are employing data to identify patterns and find solutions to the many challenges raised by the ongoing pandemic.
Duncan Watts, the Stevens University Professor in the School of Engineering and Applied Science, Annenberg School for Communication, and Wharton School
Seemingly subtle differences in individual-level human behavior—whether you stay in your house, leave and go for a walk, get on a train, go to work—all have profound consequences for how COVID-19 spreads. If you want to model that, it’s helpful to have granular, individual-level movement data. We’re working on doing that exact thing, taking standard epidemiological models and inferring very detailed networks, breaking down the city of Philadelphia into small chunks, then estimating contact rates between groups based on individuals visiting individual locations. Then we can run these models forward and try all kinds of control strategies on future caseload data.
This type of epidemiological modeling is a dramatic leap forward. Before, researchers would create these complex, agent-based models, but they would have to use indirect data like airline traffic or school attendance. Now, you have data on real people moving around. You can see what they’re actually doing and how their behavior changes when lockdown policies go into place. It’s drastically improving our ability to model the spread of disease and could have profound consequences for future pandemic response.
What happened this year with COVID could’ve happened in 2003 with SARS and any given year in between. The threat of novel viruses jumping from animals to humans is something we’ve been worrying about for decades. It turns out, we were really right to be worried and maybe we weren’t worried enough. It’s really important to do the best possible job we can of using models to make predictions and then using those to design optimal policies.
Continue reading at Penn Today.