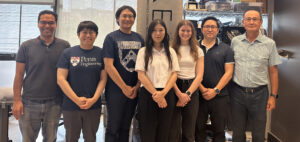
It may not always be apparent to the naked eye, but your yearly ophthalmology exams are at the forefront of two emerging trends in health care: artificial intelligence (AI) and precision medicine, where treatment is tailored to the individual’s genes, lifestyle and environment, among other factors.
With its large emphasis on imaging and cutting-edge technology including lasers, gene therapy and other approaches to care, ophthalmology is a natural fit for research on precision medicine and the use of AI. Research taking place at the Penn Research in Embedded Computing and Integrated Systems Engineering, or PRECISE, Center is examining how AI can be deployed to bring clinical practice to the next level, yielding powerful results that could one day even help prevent blindness. Ultimately, these techniques can also strengthen the patient-doctor relationship and clinical care by helping patients better understand their disease in an accessible way.
There are a lot of unanswered, but clinically relevant questions and scenarios that Penn Engineering’s PRECISE Center is seeking to address, such as how to assess visual acuity in non-responsive patients. Research at PRECISE also focuses on what can be accomplished in the health care sector using generative AI, which is AI that creates new content, including text, audio and videos, and large language models (LLMs).
“Generative AI can enhance patient-clinician interactions by providing visual aids and simulations that help patients better understand their conditions and treatment options, which in turn fosters greater trust, stronger communication and more empowered patients who feel confident in actively participating in their own care,” says Insup Lee, Cecilia Fitler Moore Professor in Computer and Information Science (CIS) and Director of PRECISE. “LLMs could enhance patient engagement and support, including providing personalized information to patients and decision support to doctors by analyzing medical records and extracting valuable insights. A key challenge for researchers will be aligning these technologies with human expertise and clinical judgment.”
Below are four projects focused on the use of AI in ophthalmology that are currently underway in the PRECISE Center.
Improving Vision Tests on Vulnerable Populations
Deep learning and AI have the potential to revolutionize health care, but it’s critical that they be safe and also robust enough to help a wide spectrum of patients, according to PRECISE Center postdoctoral researcher Kuk Jin Jang, who works in the labs of Insup Lee and Joan O’Brien, Harold G. Scheie-Nina C. Mackall Research Professor of Ophthalmology in Penn’s Perelman School of Medicine. Jang’s recent research in the ophthalmology field has focused on improving assessments of visual acuity (sharpness of vision) with state-of-the-art (SOTA) models that can provide more detailed and trustworthy data. In particular, Jang’s research focuses on uncertainty quantification and improving robustness in SOTA models to enable safe and reliable performance across patient populations.
“Our work intends to help more vulnerable populations, for which standard techniques of visual assessment may be difficult or impossible,” Jang said. “For instance, a patient who is injured and unconscious may be unable to undergo the standard visual acuity assessment procedure. Additionally, predicting treatment outcomes will not only aid clinicians in assessing risk, but also help patients make more informed decisions about treatment.”
Developing Machine Learning as an Alternative Way of Measuring Vision
As mentioned above, visual acuity (VA) is a measure of the ability to distinguish shapes and details of objects at a given distance. It is one of the first basic tests performed when an eye disease develops.
Since the existing method of measuring vision requires the patient to have a conversation with a VA technician, it is currently impossible to measure vision if patients are unconscious. Additionally, in situations such as the recent COVID-19 pandemic, where social isolation is prolonged, visiting hospitals may become difficult for patients. This makes it challenging to monitor a patient’s vision via traditional methods.
Claire Kendell, a Ph.D. student studying in PRECISE in the Lee Lab, is researching methods to analyze visual acuity for the different types of refractive errors, including myopia (nearsightedness), hyperopia (farsightedness) and astigmatism.
“Vision is one of the basic health indicators closely related to a person’s quality of life,” says Kendell. “My hope is to eventually develop machine learning and computer vision techniques to measure visual acuity using fundus images for patients with mobility difficulties, those in unconscious states, or those who would be unable to cooperate with the test, such as an infant or very young patient.”
Using Multimodal AI to Detect Abnormality or Disease
Cerebral vision impairment (sometimes called cortical visual impairment, or CVI) is a disorder caused by damage to parts of the brain that process vision. It’s a leading cause of vision loss among children in the United States, but can be difficult to detect because it’s caused by a problem with the brain, not the eyes.
Research by fellow PRECISE Center Ph.D. student and Lee Lab member Jean Park aims to use machine learning and AI to make it easier for doctors to detect eye abnormalities or diseases (such as CVI). Park’s initial focus will be utilizing machine learning algorithms on the fundus image. Complementary to fundus imaging is optical coherence tomography (OCT), a high-resolution imaging technique used to visualize and analyze the structures within the eye. Their combined use enhances diagnostic capabilities and provides a more comprehensive assessment of a spectrum of eye conditions.
By training an AI system to read the wide-field information from fundus imaging along with the detailed structural analysis from OCT, ophthalmologists can make more accurate diagnoses and monitor the progression of various eye conditions effectively, such as in age-related macular degeneration. This could also aid in surgical planning, such as in identifying epiretinal membranes in cases of retinal surgery.
“By combining different sources of information, we provide a multidimensional analysis of retinal health conditions,” says Park. “This work will add confidence for ophthalmologists to reasonably explain the decision-making process on different or multiple diagnostic modalities.”
Developing Trustworthy AI and Machine Learning Systems
Creating AI applications that are trustworthy has been a top priority surrounding its use in many industries, but it’s especially critical in health care, where lives are literally at stake.
PRECISE Center Ph.D. student Shuo Li works in the Lee Lab focusing on creating AI and machine learning systems that deliver dependable and accurate results, even when answering highly complicated questions. Li’s research employs a method called conformal prediction, which utilizes past experience to quantify the level of confidence for new predictions and can therefore dramatically increase the dependability of the performance of the AI systems.
“With more SOTA image classification models, we can get better prediction performance, and more interpretable results,” Li said. “By applying uncertainty quantification techniques to ophthalmic research, we can build more trustworthy medical applications.”
As an example of how this research could help patients or ophthalmologists, Li notes that glaucoma is five times more prevalent and up to 15 times more severe among African ancestry populations, but due to many factors, African Americans are less likely to get regular eye exams, which are critical to early diagnosis of disease.
Early detection and treatment of glaucoma is essential, as vision loss from the disease is irreversible. However, because the disease is asymptomatic in early stages, almost 50% of glaucoma patients are unaware they have the disease.
“Earlier medical intervention in glaucoma has been observed to preserve vision, and thus quality of life, for patients” says Li. “Glaucoma is a disease that most frequently effects peripheral (indirect) vision, so that may not be recognized until it is in a much more advanced phase where some of the damage is irreversible. Therefore, early detection is of utmost importance. Our work could provide a cost-effective way for individuals to detect possible glaucoma by allowing for an ophthalmology referral from home. This may increase the chance of earlier detection and intervention.”
“While AI has huge potential to improve healthcare, there are significant obstacles to ensuring that everyone realizes these benefits,” says Osbert Bastani, Assistant Professor in Computer and Information Science. “We often have lower-quality data for economically disadvantaged patients due to data collection challenges, although these patients are precisely the ones that most need help. A major goal of our work is to overcome these challenges and build models that work well for everyone.”
“We are currently developing a deep learning model that achieves high accuracy in diagnosing glaucoma from fundus photography in a large African ancestry population,” explains O’Brien. “There is a need for larger and more diverse studies on deep learning algorithms in glaucoma that utilize fundus photography, which is inexpensive and portable.”
In addition to Li’s work, Ph.D. student Sooyong Jang is developing confidence calibration techniques to assess the trustworthiness of decisions made by AI models in safety-critical settings.
Typically, confidence scores are assigned to AI model predictions. “Ideally, we would like higher confidence scores to mean the prediction is likely correct. However, such confidence outputs are generally over-confident, making them an unreliable indicator of accuracy,” says Jang. “Our confidence calibration technique will show substantial evidence to predict correctness. By quantifying the uncertainty in the predictions, ophthalmologists can then have more confidence in trusting the algorithmic outcome to complement their clinical judgement and decision making.”
If you have a research question, are interested in working with the PRECISE Center on any of its ophthalmic projects, or are an undergraduate who is passionate about the intersection of medicine and technology, email the Center at precise@seas.upenn.edu