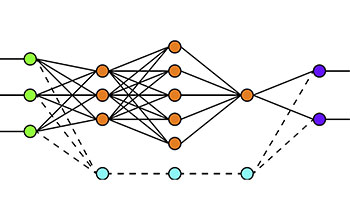
The field of artificial intelligence (AI) promises to revolutionize nearly every industry and area of science. Some AI applications are already infused into daily life, helping us to search the web, showing us the best driving route, or recommending music. These systems depend on deep learning and artificial neural networks — computer systems that digest large amounts of raw data inputs in order to train an AI without direct human supervision — yet there is a dearth of knowledge about how these systems operate on a theoretical level.
As AI-backed technologies are increasingly involved in high-level, high-stakes decisions, a more fundamental understanding of how they work is necessary.
To address this need, the U.S. National Science Foundation and the Simons Foundation Division of Mathematics and Physical Sciences have partnered to fund research through the Mathematical and Scientific Foundations of Deep Learning (MoDL) program.
As a part of this initiative, researchers at Penn Engineering, Penn Arts & Sciences and the Wharton School will partner with an interdisciplinary team led by researchers at Johns Hopkins University in a project known as Transferable, Hierarchical, Expressive, Optimal, Robust, and Interpretable NETworks (THEORINET). The collaboration will receive $10 million over five years.
Working under the conviction that technologies realize their full potential only when they are understood at a fundamental level, THEORINET researchers will build mathematical and statistical theories to explain the success of existing deep learning architectures. They expect that their work will also lead to novel machine learning techniques that will expand the reach of existing approaches.
René Vidal, director of the Mathematical Institute for Data Science and the Herschel L. Seder Professor at Johns Hopkins University, will lead THEORINET’s team of engineers, mathematicians, and theoretical computer scientists. Along with JHU and Penn, these researchers hail from multiple institutions, including the Technical University of Berlin; Duke University; the University of California, Berkeley; and Stanford University.
Penn’s contingent is led by Alejandro Ribeiro, Professor of Electrical and Systems Engineering, who focuses on the theoretical foundations of wireless networks. He will be joined by Penn Integrates Knowledge Professor Robert Ghrist, Andrea Mitchell University Professor of Mathematics and Electrical and Systems Engineering, whose research operates at the intersection of applied mathematics, systems engineering and topology; George Pappas, UPS Foundation Professor of Transportation and chair of Electrical and Systems Engineering, whose research focuses on control systems, robotics, formal methods and machine learning for safe and secure autonomous systems; and Edgar Dobriban, Assistant Professor of Statistics in the Wharton School, with expertise in the efficient statistical analysis of big data and theoretical analysis of modern machine learning and deep learning.
“NSF and our partners at the Simons Foundation recognize the importance of discovering the mechanisms by which deep learning algorithms work,” says Juan Meza, director of the Division of Mathematical Sciences at NSF. “By understanding the limits of these networks, we can push them to the next level.”
“For all of their success, the practical applicability of deep learning is still a fraction of its potential,” says Ribeiro. “There are scores of problems where deep learning could be applicable but so far has not.”
A particular focus of the Penn team is on the development of deep learning and artificial intelligence for physical systems. Theoretically grounded, principled approaches are particularly important in such systems, given their applications in medical, transportation and manufacturing settings. Those theoretical foundations are crucial for providing guarantees that a system will operate correctly in the wide variety of scenarios it might encounter, and if it does fail, it does so as safely as possible.
“AI in physical systems is different from AI in the virtual world,” says Ribeiro. “The price of failure is steep and it is only a foundations-based AI that can mitigate the risks of unexpected outcomes.”
“Receiving this center grant provides great visibility to Penn,” says Pappas. “It cements our place as one of the top destinations in the world for research in the mathematical foundations of artificial intelligence.”
Another goal for the project is to train a new, diverse STEM workforce with data science skills that are essential for the global competitiveness of the U.S. economy. This will be accomplished through the creation of new undergraduate and graduate research programs focused on the foundations of data science that include a series of collaborative research events. Women and members of underrepresented minority populations also will be served through an associated NSF-supported Research Experience for Undergraduates (REU) program in the foundations of data science.