When it comes to supercomputers, raw speed is no longer enough to tackle some of the world’s most pressing problems. When the data being crunched comes in the form of complex networks — such as tracking the billions of contacts between individuals for global COVID contact tracing — supercomputers that excel at a technique known as “graph processing” are necessary.
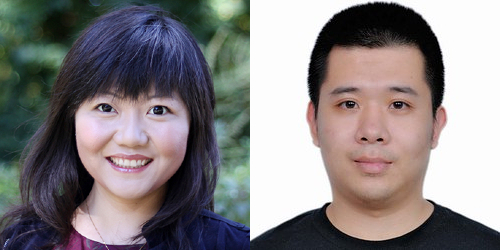
It would take the average computer years, if not decades, to fully understand the path of the COVID pandemic as it spread over the world. Worse, processing such a graph would use hundreds of megawatts, enough electricity to power Philadelphia’s homes for a year.
As graph analysis becomes integral to more and more applications, such as drug discovery, climate simulation and social science, researchers have the paradoxical task of making these supercomputers faster while using less power.
Now, researchers at the University of Pennsylvania’s School of Engineering and Applied Science have shown that their supercomputer, ENIAD, is among the best in the world when it comes to energy-efficient graph-solving.
ENIAD’s performance was certified by Graph500, the de facto standard for ranking the performance and energy efficiency of supercomputers around the world. Running one of Graph500’s benchmark graph analytics applications, ENIAD took the top spot among a list of 500 of the most energy-efficient supercomputers reported in the world.
Despite being designed and constructed by a two-person research team, ENIAD’s only close competition was Tianhe-3, China’s next-generation, exascale supercomputer, which is the product of thousands of scientists and engineers.
The researchers behind ENIAD are Jialiang Zhang, a graduate student in the Computational Intelligence lab and Jing Li, Eduardo D. Glandt Faculty Fellow and associate professor in the Department of Electrical and Systems Engineering. They named their supercomputer after ENIAC, the world’s first digital computer, which was developed at Penn 75 years ago in the same building where Li and Zhang currently work.
“We’re proud to be carrying on ENIAC’s legacy by setting this new world record,” Li says.
According to the Graph500 benchmark specification, the performance of the supercomputers is measured in MTEPS, or millions of traversed edges per second. When comparing energy efficiency, that number is divided by the number of watts of electricity used in the process of solving a standard type of network known as a Kronecker graph.
For graphs at the scale of 64 million nodes, ENIAD performed at 6,028.85 MTEPS/W, besting Tianhe-3’s 4,724.30 MTEPS/W. At this rate, ENIAD would reduce the power consumption for processing the world’s COVID contact tracing graph from hundreds of megawatts — on the scale of an entire city’s electricity usage — to several kilowatts, or what it takes to power the HVAC system of a typical household.
“Graph solving is a lot more challenging than just the regular computation that you see in most AI and machine learning applications, since you can’t parallelize it well and go faster just by adding more processors,” says Li. “But that opens up new opportunities for small-team academic researchers like us. We can have a bigger impact by exploiting a richer set of ideas than the largest industrial developers, who are likely more constrained and not looking as comprehensively at the design space.”
Rather than a challenge, Li and Zhang feel more out-of-the-box thinking beyond the abstraction levels defined by conventional computer systems was part of their recipe for success.
“We rethought the ENIAD design entirely from the ground up for AI and big data,” says Zhang. “Supercomputer hardware and software are often developed in isolation from one another based on a fixed abstraction, so the fact that we were able to co-design the hardware and software through a set of new abstractions was one of our secret ingredients.”
Li and Zhang also believe that these records are just the beginning for ENIAD. They will present new performance results for ENIAD at the Hot Chips conference next month.