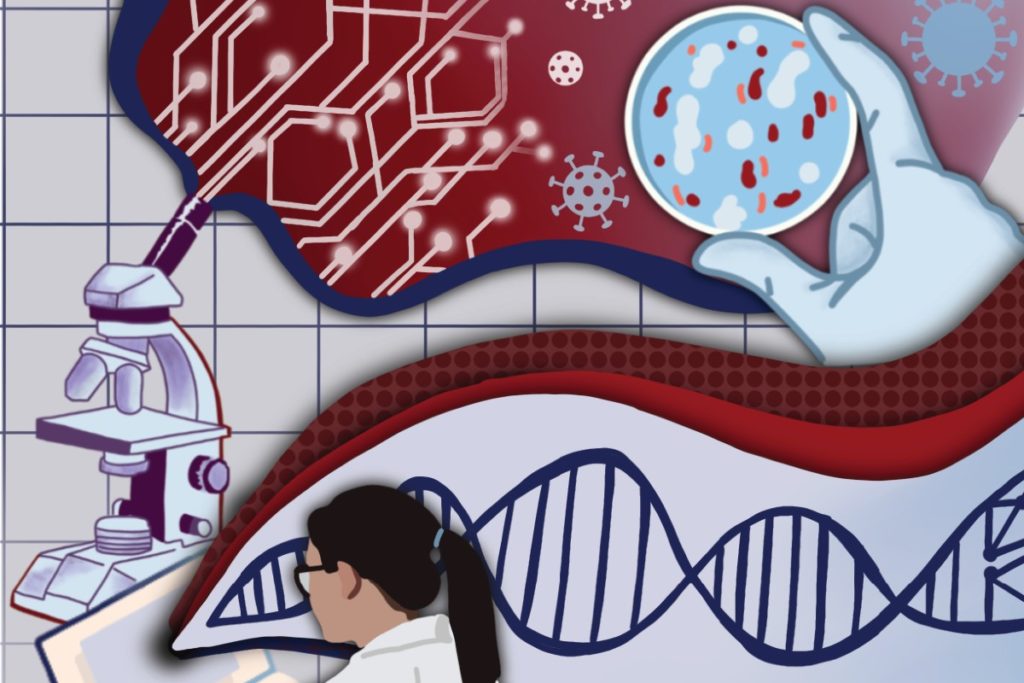
Penn Engineering’s newly established ASSET Center aims to make AI-enabled systems more “safe, explainable and trustworthy” by studying the fundamentals of the artificial neural networks that organize and interpret data to solve problems.
ASSET’s first funding collaboration is with Penn’s Perelman School of Medicine (PSOM) and the Penn Institute for Biomedical Informatics (IBI). Together, they have launched a series of seed grants that will fund research at the intersection of AI and healthcare.
Teams featuring faculty members from Penn Engineering, Penn Medicine and the Wharton School applied for these grants, to be funded annually at $100,000. A committee consisting of faculty from both Penn Engineering and PSOM evaluated 18 applications and judged the proposals based on clinical relevance, AI foundations and potential for impact.
Artificial intelligence and machine learning promise to revolutionize nearly every field, sifting through massive amounts of data to find insights that humans would miss, making faster and more accurate decisions and predictions as a result.
Applying those insights to healthcare could yield life-saving benefits. For example, AI-enabled systems could analyze medical imaging for hard-to-spot tumors, collate multiple streams of disparate patient information for faster diagnoses or more accurately predict the course of disease.
Given the stakes, however, understanding exactly how these technologies arrive at their conclusions is critical. Doctors, nurses and other healthcare providers won’t use such technologies if they don’t trust that their internal logic is sound.
“We are developing techniques that will allow AI-based decision systems to provide both quantifiable guarantees and explanations of their predictions,” says Rajeev Alur, Zisman Family Professor in Computer and Information Science and Director of the ASSET Center. “Transparency and accuracy are key.”
“Development of explainable and trustworthy AI is critical for adoption in the practice of medicine,” adds Marylyn Ritchie, Professor of Genetics and Director of the Penn Institute for Biomedical Informatics. “We are thrilled about this partnership between ASSET and IBI to fund these innovative and exciting projects.”
Seven projects were selected in the inaugural class:
Neurosymbolic approach for predictive modeling of adverse events from EHR data
Mayur Naik, Professor, Department of Computer and Information Science, Penn Engineering
Ravi B. Parikh, Assistant Professor, Departments of Medical Ethics and Health Policy and Medicine, Penn Medicine
The problem of predicting adverse events for patients from labeled electronic health record (EHR) data has many important applications in healthcare. This project will develop a novel neurosymbolic approach that harnesses the benefits of both deep neural networks and traditional interpretable models without suffering their limitations. The key insight is to model the process of predicting an adverse event as a sequential decision-making problem, mimicking the diagnosis process of a clinician. The project will focus on the application of prediction of 180-day mortality among patients with cancer, which is a commonly accepted high-risk marker. However, the proposed approach is general and will be applicable to predicting other kinds of adverse events such as disease prognosis, unplanned hospital readmission, complications in pregnant women, and many others.
Optimizing clinical monitoring for delivery room resuscitation using novel interpretable AI
Elizabeth Foglia, Associate Professor, Department of Pediatrics, Penn Medicine and the Children’s Hospital of Philadelphia
Dani S. Bassett, J. Peter Skirkanich Professor, Departments of Bioengineering and Electrical and Systems Engineering, Penn Engineering
This project will apply a novel interpretable machine learning approach, known as the Distributed Information Bottleneck, to solve pressing problems in identifying and displaying critical information during time-sensitive clinical encounters. This project will develop a framework for the optimal integration of information from multiple physiologic measures that are continuously monitored during delivery room resuscitation. The team’s immediate goal is to detect and display key target respiratory parameters during delivery room resuscitation to prevent acute and chronic lung injury for preterm infants. Because this approach is generalizable to any setting in which complex relations between information-rich variables are predictive of health outcomes, the project will lay the groundwork for future applications to other clinical scenarios.
Trustworthy explainable AI to revolutionize breast cancer risk assessment with digital breast tomosynthesis
Despina Kontos, Matthew J. Wilson Professor of Research Radiology II, Department of Radiology, Penn Medicine
Lyle Ungar, Professor, Department of Computer and Information Science, Penn Engineering
Accurate breast cancer risk assessment is critical for implementing tailored screening and prevention strategies. However, current risk assessment tools have only modest discriminatory accuracy, being limited in guiding personalized clinical decisions. Combining expertise in computational breast imaging and cancer risk assessment with deep learning and explainable AI, this partnership will develop novel, explainable and trustworthy AI approaches that will pave the way for next-generation breast cancer risk assessment models in the clinic and towards further personalizing breast cancer screening and prevention.
Utilizing deep learning to diagnose glaucoma from fundus photography in African ancestry individuals
Joan O’Brien, Harold G. Scheie-Nina C. Mackall Research Professor, Department of Ophthalmology, Penn Medicine
Osbert Bastani, Assistant Professor, Department of Computer and Information Science, Penn Engineering
This project will develop a deep learning model that accurately diagnoses glaucoma from ophthalmic images. Glaucoma is the leading cause of irreversible blindness worldwide, and more than 50 percent of people are unaware they have the disease. As the prevalence of glaucoma continues to rise in an aging population, there is a growing mismatch between the need for accurate diagnostic tests and the supply of available resources and ophthalmologists. The team seeks to develop AI that recognizes the presence of glaucoma on ophthalmic images, allowing referral of patients to an ophthalmologist for a full examination and treatment. This system has the potential to greatly increase access to care and lessen the burden on healthcare systems, ultimately helping to prevent irreversible blindness from glaucoma. The team will begin the development of the deep learning model with images from an African ancestry population, as these individuals are disproportionately affected by glaucoma, yet remain understudied.
Multimodal explainable AI for prognostic stratification of glioblastoma patients
Spyridon Bakas, Assistant Professor, Departments of Pathology and Laboratory Medicine and Radiology, Penn Medicine
Sharath Chandra Guntuku, Research Assistant Professor, Department of Computer and Information Science, Penn Engineering
MacLean Nasrallah, Assistant Professor, Department of Pathology and Laboratory Medicine, Penn Medicine
This project will lead the development of autotuned machine learning algorithms to learn patterns of prognostic relevance spanning across phenotypical characteristics, demographics, and social determinants of health of glioblastoma patients by integrating digitized brain tumor tissue sections and patients’ electronic health records. Glioblastoma is the most common aggressive brain tumor and this multi-modal interpretable approach is aimed at improving the treatment team’s decision-making.
Calibrated machine learning methods for mobile health intervention
Ian Barnett, Assistant Professor, Department of Biostatistics in Biostatistics and Epidemiology, Penn Medicine
Edgar Dobriban, Assistant Professor, Department of Statistics and Data Science, Wharton
Pratik Chaudhari, Assistant Professor, Department of Electrical and Systems Engineering, Penn Engineering
This project will develop calibrated machine learning methods to predict adverse psychiatric events in mobile health contexts. This research will develop novel regularization methods to efficiently calibrate machine learning methods trained via smooth optimization. These will be applicable to state-of-the-art neural nets, and they will be more sample-efficient than existing post hoc calibration methods. These methods will be applied to machine-learning models based on multi-modal digital biomarkers of behavior derived continuously from passively collected smartphone sensor data and will be used to prompt timely interventions for patients with psychiatric disorders.
Personalized medicine for hyperintensive pregnancy disorders
Paris Perdikaris, Assistant Professor, Department of Mechanical Engineering and Applied Mechanics, Penn Engineering
Walter R. Witschey, Associate Professor, Department of Radiology, Penn Medicine
Nadav Schwartz, Associate Professor, Department of Obstetrics and Gynecology, Penn Medicine
Combining computational fluid dynamics, machine learning and magnetic resonance imaging, this project will create digital, patient-specific “fingerprints” that describe the response of hypertensive patients to a family of pressure wave-forms under many possible flow conditions. The computational infrastructure developed in this project aims to provide trustworthy predictions of strong biomarkers for early diagnosis of hypertensive pregnancy disorders in a real-time clinical setting.