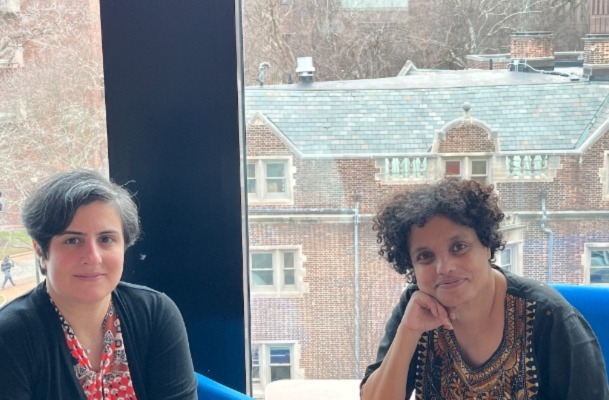
If it were up to engineering, everything would be optimized. Efficiency would rule, and the best available outcomes would frictionlessly elevate scientific research, live at our fingertips and shape our surroundings.
But engineering is a field of inquiry rooted in practice. Its ideals are brought to life with tenacity and care by engineers, who ground aspirations for progress in the messiness of what’s possible.
Shirin Saeedi Bidokhti and Saswati Sarkar are electrical engineers. They analyze and design networks that knit together a world of Internet-enabled devices, creating intelligent infrastructures that send and receive information with reliability, security and speed.
Yet their interest in connectivity is not limited to the Internet of Things. Together, they have produced a suite of studies that apply techniques from network and information theory to pandemic control and prevention.
Designed in partnership with medical experts, these tools, developed originally to boost the performance and safety of wireless objects, prove to be flexible and rigorous enough to care for networks of people. Bidokhti and Sarkar’s findings hold the potential to deliver life-saving benefits while accommodating the most human of constraints, including unequal access to medical resources, reluctance, budgetary constraints, testing errors and local practices.
Specialists in transmission, Bidokhti and Sarkar initiated these projects in response to the Covid-19 pandemic, leveraging similarities between how information travels through networks and how illness moves through populations. Their computational techniques and data-driven strategies offer insights for minimizing disease spread that improve on state-of-the-art practices in epidemiology.
The research outlines tactics to make testing, vaccination and quarantine more precise, cost-efficient and equitable, with evidence pointing to significant benefits through straightforward adjustments to public health approaches.
Having disseminated their work at a conference hosted by the Centers for Disease Control and Prevention (CDC) and authored peer-reviewed studies published and forthcoming in journals in the biological sciences to machine learning, the value of their research is clear. Next steps aim at collaboration with policymakers, practitioners and the public to adapt the research into reality.
What questions would a network theorist ask to end a pandemic? The perspective is a unique one, yielding solutions that are both optimized and human-centric.
How many hops?
Public health testing strategies rely on contact tracing. When someone tests positive for a contagious disease, their interactions are mapped and tested. But unless one of those contacts tests positive as well, contacts of contacts are not part of the initial testing equation. Meanwhile, the disease may be spreading among and beyond these first-degree contacts, symptomatically or asymptomatically, and contributing to dangerous, even explosive, spread. How do we know how many degrees of separation to test so that the time and cost pay off?
We developed a new testing strategy inspired by wireless “multi-hop communication” strategies that efficiently route signal and information from device to device. Our algorithm can figure the ideal number of “hops” from a patient to test so that a disease is contained straightaway, with benefits that can be verified to outweigh the cost.
We can see, for example, with Covid-19, that testing two or three hops shows a significant improvement in containing disease over traditional contact tracing. But the amazingly stark phase transitions in the data show that four or five is overkill! Two- or three-hop testing may involve greater cost up front, but our findings show that these strategies maintain or decrease the overall number of tests needed, while significantly reducing the spread of disease.
Explore or exploit?
Our research aspires to eliminate the need for massive lockdowns. To really maximize disease containment, we need to reconfigure the way we understand networks of spread.
Multi-hop contact tracing is a practical solution that quickly identifies people testing positive so they can be treated and quarantined. It also contributes to our data for analyzing the likelihoods of infection for people within the larger population. If we only have a certain number of tests, we need to make sure that we are using them on people with the highest probability of being infected.
But to be as effective as possible, we also need to make sure that these probabilities are as accurate as possible. We may think a particular person or group is highly likely to be infected, but what we learned is that blind spots remain in the network, even with the multi-hop testing data.
This is because we had been focusing on exploiting—contact tracing and testing—rather than exploring—testing outside the known network. What we learned is that exploration is necessary with diseases like Covid-19 that spread asymptomatically. With exploration, we really have no direct reason to think anyone is infected. But because the infection may be spreading silently at dangerous rates, we developed tools to refine exploration as a testing strategy that allows for our model to accommodate living probabilities, updating as our infection mapping becomes more precise. We can calculate, circumstance to circumstance, when exploitation is merited and when it is worth to wait to test until exploration has been conducted.
High risk or high contact?
Most of us remember the priority grouping from the initial Covid-19 vaccine rollouts. The populations at highest risk for the worst outcomes of the disease were vaccinated first, followed by those whose profession or lifestyle put them in contact with large groups of people.
As network experts, we attend to variety and efficacy within a network in a much more precise way. Public health models tend to take the homogeneity of the infection network for granted—that is, they weight each person and each path between these people in relatively similar terms. But we can provide tools with more dimension to them. When the speed of a vaccination rollout is crucial or when the number of vaccines is limited—as it often is around the world—policymakers and practitioners need to know the optimal order in which to vaccinate to minimize spread.
We include not only the variables of risk and contact levels, but their overlaps—high risk/high contact, low risk/low contact, high risk/low contact, and low risk/high contact. We can factor in the disease’s rate of spread and social and economic factors to protect the most vulnerable populations.
Say, for example, you have two individuals. One is elderly, at high risk for severe illness. The other is young and healthy but working as a taxi driver and therefore in contact with exponentially more people. The elderly person has a greater chance of complication should they fall ill, but the healthy younger person may be spreading the disease at a rate that merits they be given priority for vaccination. In other words, vaccinating the taxi driver first may be the best way to protect the elderly person.
Our research shows that the right choice is sometimes counterintuitive, and it can change along with the variables of the disease and populations. The tools we offer help practitioners protect a maximum of people by making these decisions in resource-efficient, data-informed ways.
Shirin Saeedi Bidokhti is Assistant Professor in the Department of Electrical and Systems Engineering, with a secondary appointment in the Department of Computer and Information Systems.
Saswati Sarkar is Professor in the Department of Electrical and Systems Engineering.
Collaborators include Harvey Rubin, Professor of Medicine at the University of Pennsylvania, Raghu Arghal, Xingran Chen, Jungyeol Kim and Hesam Nikpey.
This work is supported by National Science Foundation grants NSF-2047482, NSF-1910594 and NSF-2008284.