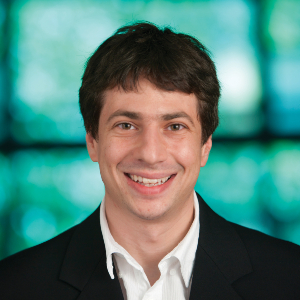
By picking out hidden relationships within vast data sets, machine learning algorithms promise to revolutionize entire industries with faster, more accurate decisions and personalized recommendations.
With public concerns about the relationship between machine learning and privacy growing, however, WIRED reporter Tom Simonite spoke with Aaron Roth, professor in the Department of Computer Science, about the emerging field of “machine unlearning.”
Roth, co-author of The Ethical Algorithm, is one of the leading figures in the field of differential privacy, which aims to preserve the power of machine-learning-based systems while preventing individual data from being teased out of the aggregate.
Although intuitive to anyone who has rued what they shared online, that notion of artificial amnesia requires some new ideas in computer science. Companies spend millions of dollars training machine-learning algorithms to recognize faces or rank social posts, because the algorithms often can solve a problem more quickly than human coders alone. But once trained, a machine-learning system is not easily altered, or even understood. The conventional way to remove the influence of a particular data point is to rebuild a system from the beginning, a potentially costly exercise. “This research aims to find some middle ground,” says Aaron Roth, a professor at the University of Pennsylvania who is working on machine unlearning. “Can we remove all influence of someone’s data when they ask to delete it, but avoid the full cost of retraining from scratch?”
Read Tom Simonite’s “Now That Machines Can Learn, Can They Unlearn?” at WIRED.