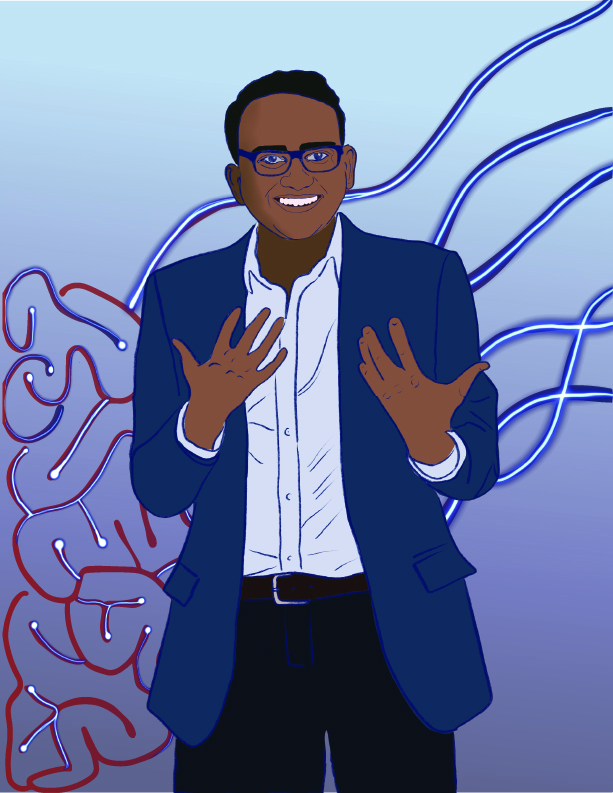
Learning is ubiquitous in nature, and the way systems adapt to new circumstances exhibits some striking similarities. At the microscopic scale, networks of proteins control how other enzymes are synthesized, determining processes ranging from the rate of cell division, to how quickly we adjust to jet lag. On a larger scale, different types of neurons in the brain, each with a specific function, organize themselves in complex patterns to process different types of information. And on an even larger scale, organisms like humans can learn many new skills without forgetting old ones.
Despite huge differences in how these systems work, there is a common theme that connects them: learning systems are robust and yet versatile. Now, Penn Engineers are working to unravel the underlying similarities between these systems with the goal of making machine learning more efficient and more widely applicable.
“We are trying to understand the fundamentals of learning,” says Pratik Chaudhari, Assistant Professor in Electrical and Systems Engineering and Computer and Information Science. “We are identifying principles and patterns that connect learning in its various forms, from biological systems to artificial neural networks that mimic how the human brain learns.”
Chaudhari is a recipient of the National Science Foundation’s CAREER Award in support of this effort. The CAREER Award is given to early-career faculty who have the potential to chart innovative research directions and are committed to education, outreach and public engagement.
“The essential functions of cellular biology continue to take place even if the concentration of proteins inside the cell changes by a lot,” says Chaudhari. “That is what we mean by ‘robust.’ But your body can also adapt to a new time zone within a few days, which is what we mean by ‘versatile.’ Circuits in the retina convert about a billion photos in a tiny flash of light into a single event for the brain to register, but the same circuits can also process the detailed spatiotemporal correlations in a video. Most of us learned to ride a bicycle as children, and even if we haven’t used one recently, we are able to relearn how to ride one quickly.”
As part of this research, Chaudhari will investigate a special property, which machine learning researchers call “sloppiness,” that allows these biological learning systems to regulate their output at multiple levels of sensitivity. Artificial learning systems known as “deep neural networks” also rely on this same property to make accurate predictions.
“For example,” Chaudhari says, “we have found that only about 0.5 percent of the millions of neurons in a typical deep network govern the predictions for a given problem. You can change the others by many, many orders of magnitude without hurting the accuracy of the predictions. At the same time, you need to change very few neurons in a deep network that can predict the next word of a sentence of English to make it predict the next word of Spanish. Just like biological systems, deep networks are neither completely robust, nor exceedingly sensitive, they are both!”
Deep neural networks are the latest incarnation of efforts spanning multiple decades to imitate learning in the human brain.
“Most technology that we use today, from word prediction when we text, to face recognition when we take a picture, to recommendations of which shoes to buy when we shop, are driven by deep learning today,” Chaudhari says. “We have built this technology very quickly and very successfully. But we do not really know why it works. If it breaks, we do not know how to fix it.”
Using this grant, Chaudhari’s group plans to study how sloppiness that seems persistent across biological learning systems can explain how deep neural networks work. His group has set their sights on a key challenge in machine learning today: how to learn effectively with small amounts of data.
One real-world application of learning with small data sets is diagnosing Alzheimer’s disease.
“The typical symptom of Alzheimer’s is dementia, but there are many possible underlying causes,” says Chaudhari. “There are also many ways to diagnose the disease, such as using radio imaging data to study the atrophy of different parts of the brain. But, of course, everyone’s brain is different, as is everyone’s genetic makeup. Additionally, there are different demographic and socioeconomic factors to consider in a diagnosis, making the entire process challenging.”
While machine learning is fundamentally about discovering salient features from data, there is no such thing as the typical patient, making such data highly heterogeneous. To make progress on these problems, scientists need to build new methods that work well with small amounts of data.
“Small data is the next frontier of machine learning,” says Chaudhari. “We need to plug in everything that we know about the problem to make progress here, from modeling the underlying biology, to our understanding of the models such as deep neural networks that we fit on such data to make predictions. If we are successful at developing these ideas, we can glean insights into many other problems in addition to Alzheimer’s.”
Chaudhari’s research will be accompanied by educational and outreach initiatives that focus on bringing the world of data science to students across high school and graduate levels at Penn and through collaborations with the Center for Teaching and Learning at Penn and the Franklin Institute. He also plans to initiate the “Greater Philly Machine Learning Day,” a one-day event that fosters collaborations across universities and industries in Philadelphia.
“Research is not something you do after studying, it is how you study,” says Chaudhari. “The educational material that I will develop with the funding from the CAREER Award will be targeted to all age levels, from projects for first-year undergraduates to more advanced material in graduate courses. It will help students get hands-on experience in developing machine learning systems and understanding the interdisciplinary principles that govern them.”