The Brain in the Machine
Insights into how computers learn, the current challenges of artificial intelligence research, and what the future holds for how machines might shape society in the future.
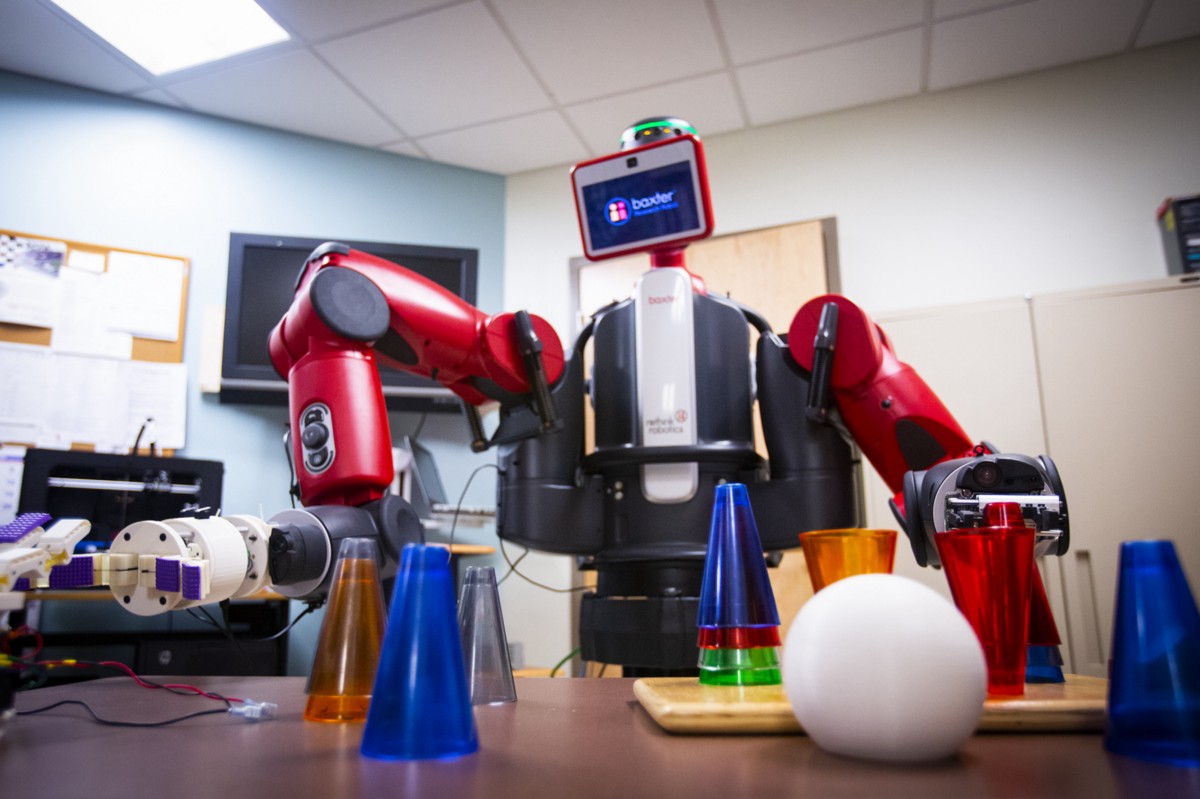
By Erica K. Brockmeier
The phrase “artificial intelligence” might conjure robotic uprisings led by malevolent, self-aware androids. But in reality, computers are too busy offering movie recommendations, studying famous works of art, and creating fake faces to bother taking over the world.
During the past few years, AI has become an integral part of modern life, shaping everything from online shopping habits to disease diagnosis. Yet despite the field’s explosive growth, there are still many misconceptions about what, exactly, AI is, and how computers and machines might shape future society.
Part of this misconception stems from the phrase “artificial intelligence” itself. “True” AI, or artificial general intelligence, refers to a machine that has the ability to learn and understand in the same way that humans do. In most instances and applications, however, AI actually refers to machine learning, computer programs that are trained to identify patterns using large datasets.
“For many decades, machine learning was viewed as an important subfield of AI. One of the reasons they are becoming synonymous, both in the technical communities and in the general population, is because as more data has become available, and machine learning methods have become more powerful, the most competitive way to get to some AI goal is through machine learning,” says Michael Kearns, founding director of the Warren Center for Network and Data Sciences.
If AI isn’t an intelligent machine per se, what, exactly, does AI research look like, and is there a limit to how “intelligent” machines can become? By clarifying what AI is and delving into research happening at Penn that impacts how computers see, understand, and interact with the world, one can better see how progress in computer science will shape the future of AI and the ever-changing relationship between humans and technology.
Intelligent machines?
All programs are made of algorithms, “recipes” that tell the computer how to complete a task. Machine learning programs are unique: Instead of detailed step-by-step instructions, algorithms are “trained” on large datasets, such as 100,000 pictures of cats. Machine learning programs then “learn” which features of the image make up a cat, like pointed ears or orange-colored fur. The program can use what it learned to decide whether a new image contains a cat.
Computers excel at these pattern-recognition tasks, with machine learning programs able to beat human experts at games like chess or the Chinese board game GO, because they can search an enormous number of possible solutions. According to computer scientist Shivani Agarwal, “We aren’t designed to look at 1,000 examples of 10,000 dimensional vectors and figure out patterns, but computers are terrific at this.”
For machine learning programs to work well, computers need a lot of data, and part of what’s made recent AI advances possible is the Internet. With millions of Facebook likes, Flickr photos, Amazon purchases, and Netflix movie choices, computers have a huge pool of data from which to learn. Coupled with simultaneous technological improvements in computing power, machines can analyze massive datasets faster than ever before.
But while computers are good at finding cats in photos and playing chess, pattern recognition isn’t “true” intelligence — the ability to absorb new information and make generalizations. As Agarwal explains, “These are not what we would call ‘cognitive abilities.’ It doesn’t mean that the computer is able to reason.”
“Most of the successes of machine learning have been on specific goals that nobody would call general purpose intelligence,” says Kearns. “You can’t expect a computer program that plays a great game of chess to be able to read today’s news and speculate on what it means for the economy.”
Continue reading at Penn Today. This story is part of Penn Today’s series on artificial intelligence.