Shivani Agarwal: Looking at Machine Learning from All Angles
by Lida Tunesi
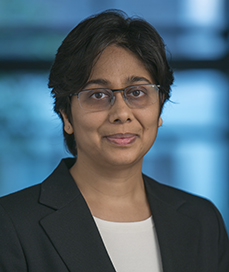
It is now an everyday occurrence to see customized recommendations while shopping online, and uncannily personalized sidebar ads while browsing a website. Both of these marketing tools are powered by machine learning, a field of study that extends to many other parts of society as well. Machine learning now powers advancements in speech recognition, drug development, detection of fraudulent transactions, self-driving cars, and myriad other applications.
“Today, machine learning has become a force of its own,” says Shivani Agarwal, Rachleff Family Associate Professor in Computer and Information Science. “Almost every application that requires discovering patterns in data or building models from data makes use of machine learning methods.”
Agarwal studies many sides of the discipline, from exploring the fundamental strengths and weaknesses of machine learning methods, to discovering its connections to other disciplines, like economics and psychology.
The goal of machine learning is for a computer to “learn” to perform a task without specific instructions. Programmers can create algorithms that take in data sets and, using that information, figure out how to find patterns or pick out certain traits. For example, an algorithm can learn to identify spam emails by analyzing examples of both spam and non-spam messages. The algorithm’s ability to correctly distinguish junk mail keeps improving as it looks at more and more examples.
Research in machine learning largely arose from the desire to have computers learn to solve increasingly complex problems in computer science, and deal with bigger and bigger data sets in statistics. Nowadays, it is the “engine” behind modern data science, Agarwal says. In other words, tools from this field are useful for any discipline that wants to turn massive piles of data into meaningful information — including disciplines that might not seem related to computer science, such as biology.
Even in the late 1990s, Agarwal says, scientists had already bridged machine learning to the life sciences. Researchers used machine learning methods to analyze newly available genomic data in order to, for instance, identify genes involved in certain diseases or find patterns in gene regulation. Since then, the connection to biology has only grown stronger.
“Today, most life sciences laboratories produce vast amounts of data that simply cannot be analyzed by hand or eye,” Agarwal says, “and machine learning methods are increasingly becoming a central part of their toolbox.”
Engaging in applications of machine learning, such as with the life sciences, is another theme of Agarwal’s research. “We collaborate with scientists and practitioners in other disciplines, and help them identify or develop machine learning methods that can be used to solve the problems they care about,” she says.
For instance, in a joint effort with startup Mitra Biotech, Agarwal used machine learning methods to predict how patients would respond to a certain anti-cancer drug. The team’s results turned out to be more accurate than current biomarker-based methods. This was good news for researchers at Mitra, but the project was valuable for Agarwal as well.
“It is important to be able to test how well our methods perform on real-life, human problems,” Agarwal says. “This collaboration both helped to solve the problem faced by my life-scientist collaborators, and helped to validate the machine learning methods we had developed.”
Experiments in the life sciences can also bring up new problems for machine learning to attempt to solve, Agarwal says. These types of challenges help to push the field forward.
“Over the years, many new machine learning methods have been developed in order to solve a data-based problem in the life sciences for which no standard method was applicable,” Agarwal says.
In her research at Penn, Agarwal also meshes machine learning with a host of other academic fields. Her group recently brought together ideas from theoretical computer science, spectral graph theory, operations research and statistics to study pairwise comparisons, a type of choice made in recommender systems and marketing. The group hopes to expand on their results to study more types of machine learning choices. Choice data, Agarwal says, is an emerging topic that sits in the overlap of machine learning and econometrics.
Some of Agarwal’s other work gets back to the fundamentals of the field. Researchers can evaluate how “good” a machine learning model is through various kinds of performance measures. If the performance measures are complex, extra thought and care must go into designing the model. Agarwal’s group has been developing design principles to help with this process, and plans to continue building on their work in the coming years. They hope to make the tools that result from the work more easily available to machine learning users.
Penn’s effort to become a leader in both the teaching and research of machine learning was, for Agarwal, a big part of the University’s appeal.
“There is a huge need for centers of excellence in machine learning across the country,” Agarwal says, “and I believe Penn is well-positioned to play a major role in this direction.”
As part of this endeavor, Agarwal co-directs Penn Research in Machine Learning, a joint effort between Penn Engineering and Wharton to bring together the University’s large and diverse machine learning community.
There were also reasons outside of academics to come to Penn.
“I am fortunate to have a very supportive set of colleagues here, as well as terrific support staff and students,” Agarwal says. “It also helps that Penn is located in Philadelphia — a historic, modern, and cosmopolitan city.”
Agarwal foresees no shortage of interesting questions and ideas to investigate in her research. Despite modern advances in machine learning, there are still some missing links between the field’s theory and practice.
“Even today, many machine learning methods are used without a clear understanding of why they work or when they might fail,” Agarwal says. “This gap motivates a lot of the work we do in my research group, and I hope we will see the gap narrow in the years ahead.”