Penn Researchers Establish Universal Signature Fundamental to How Glassy Materials Fail
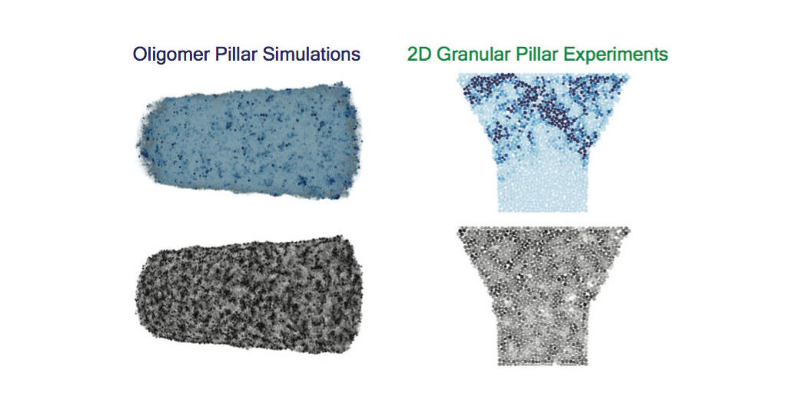
Dropping a smartphone on its glass screen, which is made of atoms jammed together with no discernible order, could result in it shattering. Unlike metals and other crystalline material, glass and many other disordered solids cannot be deformed significantly before failing and, because of their lack of crystalline order, it is difficult to predict which atoms would change during failure.
“In order to understand how a system chooses its rearrangement scenario,” said Douglas Durian, professor of physics and astronomy at the University of Pennsylvania, “we must make connection with the underlying microscopic structure. For crystals, it’s easy; rearrangements are at topological defects such as dislocations. For disordered solids, it’s a very hard 40-year-old problem that we’re now cracking: What and where are structural defects in something that’s disordered?”
To find a link between seemingly disparate disordered materials, an interdisciplinary collaboration between Penn researchers in the School of Arts and Sciences and the School of Engineering and Applied Science with expertise in various materials studied an unprecedented range of disordered solids with constituent particles ranging from individual atoms to river rocks. Understanding materials failure on a fundamental level could pave the way for designing more shatter-resistant glasses or predicting geological phenomena like landslides.
In a paper published in Science, the Penn researchers revealed commonalities among these disordered systems, defining a counterpart to the “defects” implicated in crystalline materials failure. This so-called “softness” in disordered systems predicts the location of defects, which are the collection of particles most likely to change when the material fails.
The researchers used a technique developed by Durian with Penn Ph.D. graduate Samuel Schoenholz, and Harvard University Ph.D. graduate Ekin Dogus Cubuk, both currently at Google Brain; Andrea Liu, Hepburn Professor of Physics in Penn’s School of Arts and Sciences; and Efthimios Kaxiras, John Hasbrouck Van Vleck Professor of Pure and Applied Physics, Harvard School of Engineering and Applied Sciences. Liu and Daniel Gianola, then a professor in Penn’s School of Engineering and Applied Science’s Department of Materials Science and Engineering and now at the University of California, Santa Barbara, led the study. Daniel Strickland and Robert Ivancic, both graduate students at Penn, are first authors, together with Cubuk and Schoenholz.
The paper is the culmination of years of research conducted at Penn’s Materials Research Science & Engineering Center (MRSEC) which is hosted by the Laboratory for Research on the Structure of Matter. Liu and Robert Carpick, John Henry Towne Professor and chair in Mechanical Engineering and Applied Mechanics at Penn were co-leaders of the MRSEC’s integrated research group focused on the mechanics of disordered packings.
A dozen of the group’s faculty members, along with students and postdoctoral researchers from their labs, contributed to the study, providing data from 15 simulations and experiments on different types of disordered systems. The particles in those systems ranged in size from carbon atoms that make up wear-resistant engine coatings to centimeter-sized plastic spheres in a model riverbed.
Using machine learning, the researchers collected hundreds of quantities that characterize the arrangements of particles in each system, quantities that individually might not be expected to reveal much. Importantly, they found the combination of these quantities that correlates strongly with the dynamics. This produced a microscopic structural property called softness. If softness is known, the behavior of the disordered material and how likely its constituent particles are to rearrange can be predicted.
Continue reading on Penn News.