Penn Engineers Win Award for Paper on AI for Smart Buildings
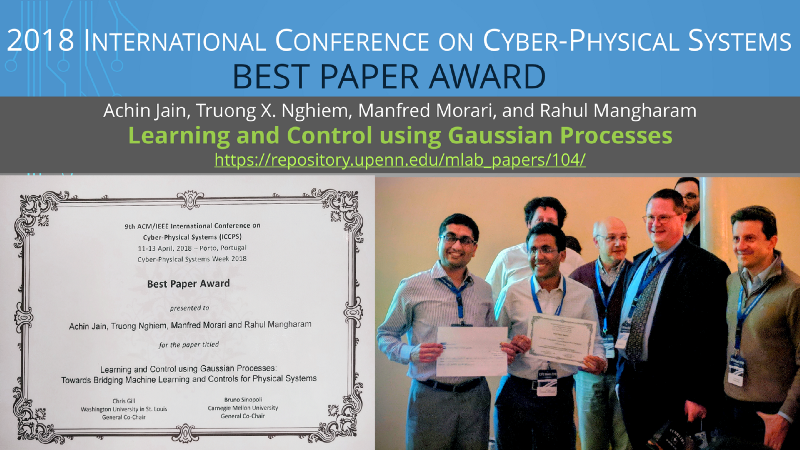
Engineering graduate student Achin Jain, professors Rahul Mangharam and Manfred Morari, and alumnus Truong X. Nghiem won the Best Paper Award at the 9th IEEE/ACM International Conference on Cyber-Physical Systems (ICCPS) 2018 for their work on bridging machine learning and control theory for physical systems. ICCPS is part of CPSWEEK, which is one of the largest conferences in the Internet of Things (IoT) field.
Their work solves a challenging and high impact problem of bridging machine learning and control of physical systems.
“Traditionally,” Mangharam says, “machine learning folks’ position has been ‘Give me data and I will give you a model that is good for prediction,’ while control systems engineers say, ‘Give me an accurate enough model and I will guarantee the performance and stability of your plant.’ The key issue is that the data-driven models captured from machine learning approaches have, in general, not been applied to control systems and are inherently not compatible with control theory.”
The Penn researchers’ paper was recognized for fundamental contributions toward bridging this gap, such that data-driven modes can be used for control of physical systems. In their study, they identify methods for improving the rate at which data-driven models can generate accurate control models.
This effort is being applied to energy management in the complex and messy world of commercial buildings. Extreme price volatility has become the new norm in our electric grids. For example, in January 2014, the east coast electricity grid experienced an 86-fold increase in the price of electricity from $31/MWh to $2,680/MWh in a matter of 10 minutes. Building additional peak generation capacity is not environmentally or economically sustainable. Thus, the focus has shifted from energy efficiency, such as replacing equipment to improve the baseline by a small percentage, to energy flexibility, which includes adapting the building’s energy consumption during peak electricity price events.
Using machine learning algorithms, the researchers can now capture whole-building and zone-level models from historical operation data with 96–98 percent accuracy.
“These models are then used not only for prediction but also for synthesizing control recommendations for building operators,” Jain says. “The low-touch and low-cost approach taps existing building hardware, such as thermostats, as well as meter data from the Building Management System. It enables Model Predictive Control with machine learning algorithms reducing the cost and time of modeling by several orders of magnitude.”
Read more details in the paper here.