“Deep Learning” Can Help Computers Understand Emotional Language
Anyone who uses social media knows that it is a powerful way of not just sharing links and pictures, but for expressing how people feel about the things they share.
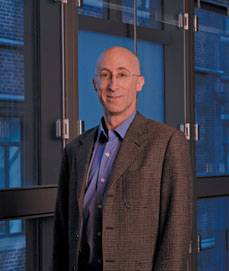
Lyle Ungar, professor in Penn Engineering’s Department of Computer and Information Science, and Muhammad Abdul-Mageed, assistant professor in the School of Library, Archival and Information Studies at the University of British Columbia, are using this fact to help computers pick those emotional cues out of text.
Better understanding the feelings behind users’ words could help digital assistants or customer service chatbots respond more appropriately and be more helpful.
At the Annual Meeting of the Association for Computational Linguistics in Vancouver, Canada last week, Ungar and Abdul-Mageed debuted EmoNet, a project that uses “deep learning” on a database of tweets to help computers correctly label the emotional tenor of a given message. The program is about as good as humans are at the task.
Deep learning is a branch of artificial intelligence inspired by how the brain processes information. Recent advances in the field show that, given enough labeled data, it should be possible to improve models that assign labels to new data.
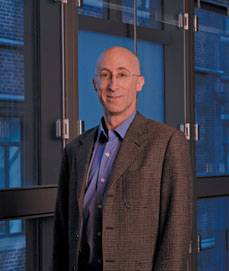
To reach this critical mass, the researchers built their database by collecting tweets that contained hashtags with words related to the 24 categories on Plutchik’s Wheel of Emotions. Starting with about 250 million tweets, the researchers implemented noise-reducing rules that eliminated duplicates and those that might mislead the software. The remaining 1.6 million dependably labeled tweets were split into a training set and a testing set.
Finally, the researchers had human coders assign emotional labels to a random subset of the testing group’s tweets and saw how closely their assessments matched the program’s.
Given the inherent subjectivity of emotions, people can’t perfectly predict how a tweet will be labeled, especially working with the 24 nuanced categories on Plutchick’s Wheel of Emotions.
EmoNet’s 87 percent accuracy rate is roughly in line with how well humans perform the task.
“When we combined some closely related categories of emotions, such as ecstasy, joy, and serenity, as one happiness category — reducing the 24 emotion categories to only eight — we reached an even higher accuracy of 95 percent,” says Abdul-Mageed.
Beyond helping to create more personable computer programs, this type of research can help people to understand others’ emotions on a larger scale than ever before.
“This work is part of the World Well-Being Project, a research group at Penn that uses machine learning to enable computers to better understand people — their personalities, emotions, and mental and physical well-being — from the language they use,” says Ungar.
“Marketing professionals want to know how people feel about the products they tweet about, but doctors want to know the emotions patients feel after surgery, and therapists want to know about how their patients feel, too,” he continues. “After giving consent, they could share their Facebook posts or tweets with various providers, who could extract the emotions presented and use those as part of therapy.”