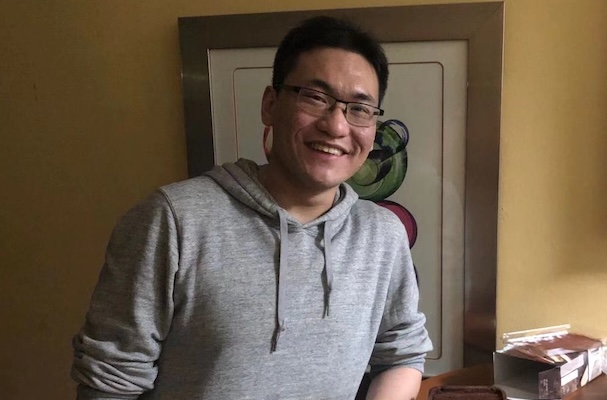
As artificial intelligence and machine learning grow in use, debate grows about how to ensure systems powered by the technology are safe to use, trustworthy in their work and efficient at performing a variety of tasks.
Shuo Li, a doctoral student in Computer and Information Science (CIS), has dedicated his research to answering these complicated questions. Li works within the Penn Research in Embedded Computing and Integrated Systems Engineering, or PRECISE, Center and is advised by Insup Lee, Cecilia Fitler Moore Professor in CIS and Director of PRECISE, and Osbert Bastani, Assistant Professor in CIS.
“The trustworthiness makes it safe to deploy AI systems, while the high efficiency enables AI systems to complete complicated tasks,” explains Li. “However, developing such systems is challenging because it is an interdisciplinary topic involving uncertainty quantification, optimization and learning theory in computer science.”
With this challenge comes collaborative opportunities, and Li’s hope is to work with experts from different backgrounds in areas such as computer science, statistics and robotics to learn and develop solutions.
His first project in this space involved developing a safe method for robots to perform tasks that included navigation and walking. Li was able to generate excellent performance from the robots using an innovative combination of reinforcement learning and predictive control, which governs the path robots will take and how they avoid obstacles.
In another project, Li used a method called conformal prediction, which employs past experience to determine levels of confidence in new predictions to control for false positives and false negatives, to develop a more trustworthy way of spotting anomalies in the performance of AI systems. “Our work was the first to provide false negative rate guarantees in the anomaly detection task,” says Li.
He also used conformal prediction in a project aimed at developing trustworthy AI systems for complicated tasks like classifying multiple types of data, object detection and answering questions based on analysis of a large collection of text sources. Li’s method combines the power of conformal prediction with research techniques that guarantee accuracy and efficiency. “We evaluated this method on various tasks, and our results showed that while guaranteeing the accuracy, our method can improve efficiency by an average of 20% over baselines,” says Li.
Li, who received a bachelor’s degree in Electronic Engineering from China Agricultural University and a master’s in the same field from Beihang University, came to Philadelphia in 2018. Before entering the doctoral program, he earned an additional master’s degree in Robotics from Penn, received an outstanding research award from Penn Engineering in 2020, and, along with fellow doctoral student at PRECISE Xiayan Ji, was a finalist in the Wells Fargo Campus Analytics Challenge. For that competition, they used a large chunk of data to develop a machine learning model for predicting elder fraud in digital payments.
“Modern large language models, or LLMs, have become enormous both in terms of the size of the model and the scale of data on which they are trained,” says Bastani. “This gives them the capability to process input text and generate output content in a way that gives the appearance of understand meaning and common sense reasoning. Despite this progress, large language models’ capability for understanding remains shallow and unreliable, leading to challenges such as hallucinations and incorrect information. Hence, it is critical for our group to develop more trustworthy AI systems based on LLMs capable of knowing their own limitations.”
The PRECISE Center’s mission is to contribute expertise to the scientific community and to train the next generation of researchers. Collaboration is essential to this work. If you have a research question, are interested in working with the Center on a project, or if you are an undergraduate who is passionate about AI and would like to work with Li on his research, email precise@seas.upenn.edu.