‘Smart Aviary’ Uses Machine Learning to Study Social Networks
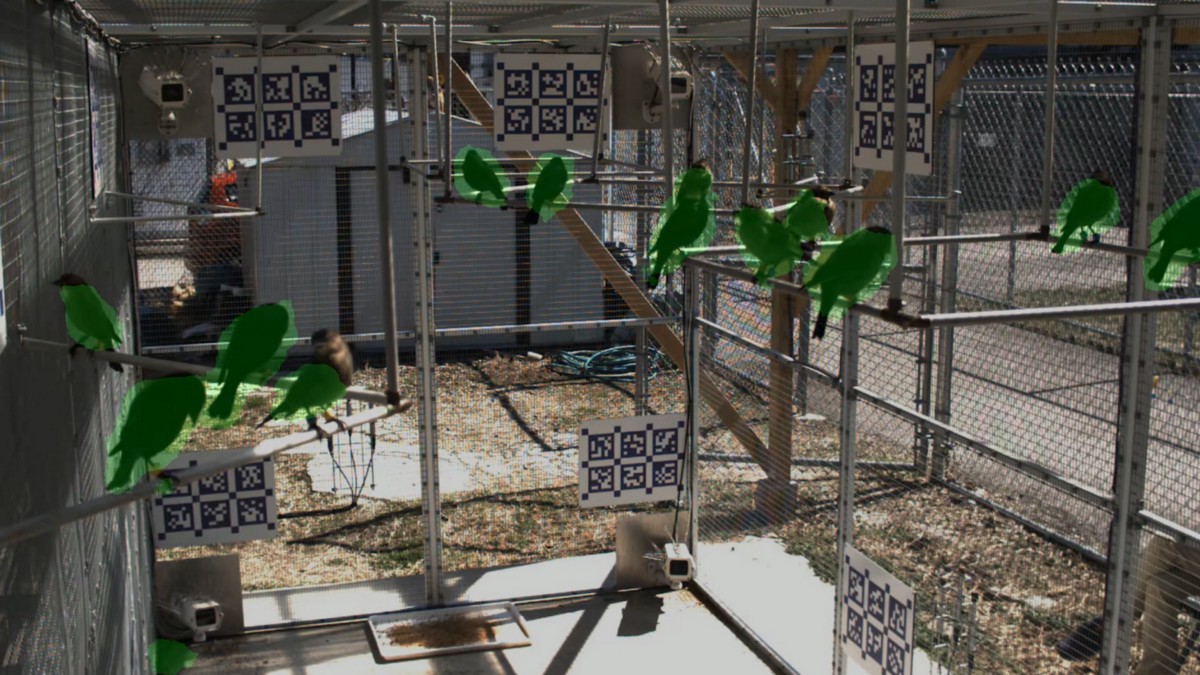
By Lauren Salig
To answer critical questions about animal behavior, scientists typically have two options: controlled laboratory experiments or temporary observation in the wild. But each option sacrifices either the accuracy of a natural environment or scientific reliability, meaning scientists struggle to paint a complete picture of how animals naturally behave over long periods of time.
In a collaboration of engineering, biology, and physics, Penn scientists have constructed a ‘smart aviary’ that combines the laboratory and the natural environment to achieve unprecedented observational ability. Located at Pennovation Works, the aviary houses 20 brown-headed cowbirds that fly around among high-tech microphones and computer-vision cameras used to track their every move. The researchers hope that analyzing data from full-time surveillance of the gregarious birds will reveal nuances in their breeding rituals and social networks that were previously impossible to detect.
Kostas Daniilidis, Ruth Yalom Stone Professor of Computer and Information Science, brought his computer vision and robot perception expertise to this project’s interdisciplinary table. With nearly constant footage of the birds coming in, Daniilidis and his lab group had to determine how to extract relevant information from massive amounts of data. That process included developing algorithms to distinguish each individual cowbird and training their machine learning programs to recognize exactly where the birds are located and note their social interactions.
In an article by Penn Today, Daniilidis also discusses the difficulty of using machine learning to recognize particular bird stances that convey important social information:
Each bird in the aviary can be distinguished by colored leg bands, but Marc Badger, a postdoctoral researcher in Daniilidis’s group, is working to craft algorithms capable of discerning different poses of the birds based on their silhouettes. Females, for example, go into what is known as a copulatory response, a kind of submissive posture, to indicate that they are receptive of a male’s advances. The engineers’ task is to employ machine learning to distinguish these types of subtle movements from others.
“It’s much easier to transfer results from joint positions in humans, or even cheetahs or monkeys, animals that have clear articulation in their joints,” says Daniilidis. “In a bird it’s very difficult to click on a point and say, ‘This is the joint of articulation,’ because a lot of articulation happens underneath the wings, for example.”
Continue reading at Penn Today.