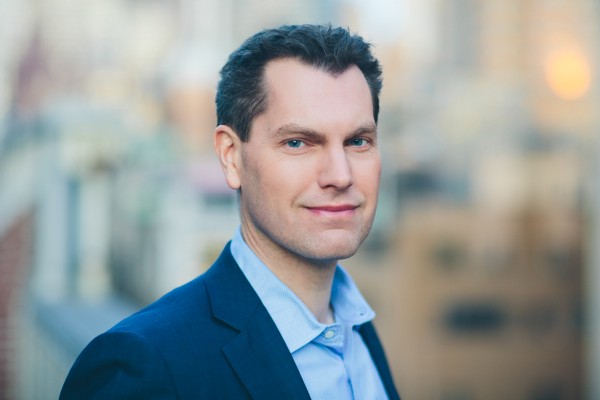
While engineers are busy inventing the future, historians are tasked with compiling records of human events so other generations may learn what came before them. But how can historians assess what present-day events will be significant to the zeitgeist of a society that is decades in the future? Duncan Watts thinks algorithms can help.
Watts, recently appointed 23rd annual Penn Integrates Knowledge University Professor, is leading computational social science research to develop a method of determining what events will be considered historically significant in the future. Watts, the Stevens University Professor, has joint faculty appointments in the department of Computer and Information Science in the School of Engineering and Applied Science, the Annenberg School for Communication, and the Department of Operations, Information and Decisions in the Wharton School. His research is focused at the intersection of sociology, math, physics, statistics, and computer and informational science, and he, along with Columbia University historian Matthew Connelly, is bringing these fields together to catch a glimpse of the future of history.
Scientific American’s Matthew Hutson recently covered this effort in an article “Automating History’s First Draft”:
Philosopher Arthur Danto argued in 1965 that even the most informed person, an “ideal chronicler,” cannot judge a recent event’s ultimate significance because it depends on chain reactions that have not happened yet. Duncan Watts, a computational social scientist at the University of Pennsylvania, had long wanted to test Danto’s idea.
Watts partnered with Connelly in analyzing a set of two million declassified State Department cables sent between 1973 and 1979, along with a compendium of the 0.1 percent of cables that turned out to be the most historically important. The data was analyzed by an AI algorithm built by Watts, and, while algorithm isn’t perfect, it far outperformed previous predictions of various cables perceived historical significance.
In a paper titled “Predicting History” and published in the journal Nature Human Behaviour, Watts and Connelly suggest that an “artificial archivist” could aid in our understanding of historical events by using the tools of data science for humanities-based applications. There is more work to be done in testing Danto’s hypothesis, but researchers agree that it is exciting to witness machine-learning tackle a “conceptual puzzle.”
Read more about Watts and Connelly’s research at Scientific American.