Penn Engineers Develop Simpler Way of Simulating Flow of Particle-laden Fluids
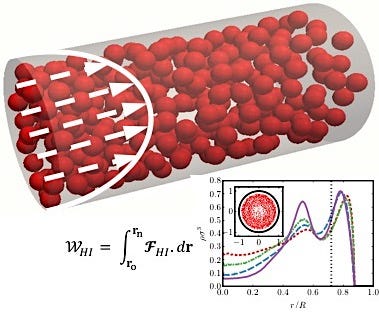
Most things that flow aren’t pure liquids; they contain solid particles with a wide range of densities and physical properties. From naturally occurring substances, like blood and molten lava, to industrial products in fields ranging from cosmetics to pharmaceuticals, these suspensions can be found everywhere.
The bumping and jostling of all of these particles — against one another and the medium itself — adds a layer of complexity when it comes to making fine-grained predictions about how suspensions will behave when they flow through a channel. Modeling the individual trajectories of each of the millions of red blood cells in a drop of blood is possible, but only supercomputers can currently handle such a computationally intense task.
In a study published in the journal Physical Review E, a team of Penn Engineering researchers laid out a theoretical framework for a much simpler way of modeling this hydrodynamic interaction.
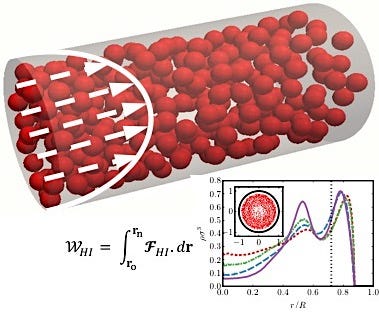
The study was led by Ravi Radhakrishnan, Professor in the Departments of Bioengineering and Chemical and Biomolecular Engineering, and Zahera Jabeen, then a postdoctoral researcher in his lab. Portonovo Ayyaswamy, Asa Whitney Professor in the Department of Mechanical Engineering and Applied Mechanics, and Hsiu-Yu Yu, a former Radhakrishnan lab member also contributed to the study. They collaborated with David Eckmann, Horatio C. Wood Professor of Anesthesiology and Critical Care in Penn’s Perelman School of Medicine.
“By developing this theoretical framework,” Radhakrishnan says, “our study enables a typical laptop to model hydrodynamic interactions for thousands of particles in flow. Previously, that was possible only by employing several hundred nodes of a supercomputer.”
These gains are enabled by focusing only on each particle’s most immediate surroundings, rather than trying to capture every long-range interaction at once.
“A flock of birds or swarm of bees fly in unison, perfectly orchestrated, but without a conductor,” Radhakrishnan says. “In essence, each bird or bee only coordinates with its nearest neighbors, carefully choosing its speed and direction to avoid collisions, and the coordination emerges automatically at the level of collective behavior. Similarly, our algorithm capitalizes on local moves for each particle of the suspension and the global coordination between all particles automatically emerges.”
The researchers’ approach involves a Monte Carlo method. This common computational technique is named after the famous Monaco casino because it relies on the probability distribution of randomly decided outcomes. Since they are based on a random search algorithm, particles’ local moves are computationally “cheap” to implement and thus vastly accelerate the speed of computation.
“Each particle moves randomly in our method, exploring the optimal configuration in its neighborhood,” Radhakrishnan says. “This enables the whole system to relax to its optimal state.”
Beyond relatively simple systems like blood or industrial slurries, future applications of this approach could include active matter, where the “particles” are complex agents capable of moving themselves in a flow. These include swarms of insects or flying robots, filaments propelled by molecular motors in biological cells, drones and robots, and self-propelled nanocarriers delivering therapeutics.
The research was supported by the National Institutes of Health through grants R01 EB006818 and U01 EB016027.