Paris Perdikaris Predicts Complex Outcomes of Physical Systems
Funding From a DOE Early Career Award Will Support Research on Computationally Intense Modeling
By Emily Schalk
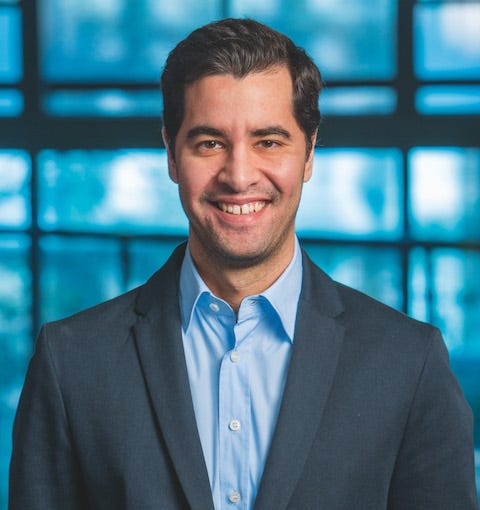
Since the days of Isaac Newton, scientists have been monitoring and predicting the movements of falling apples, heavenly bodies, ocean currents and just about anything that can go from point A to point B. These models have grown increasingly complex, but so have the physical phenomena they depict. Now the mechanisms behind cutting-edge technology, in fields ranging from aviation to nuclear waste management, can be all but impossible to fully analyze and understand.
Enter Paris Perdikaris.
An assistant professor in the Department of Mechanical Engineering and Applied Mechanics, Perdikaris is taking a new approach to modeling complex and unpredictable physical systems, research that is being funded by an Early Career Award from the Department of Energy (DOE).
The project, which was selected for funding by the DOE’s Office of Advanced Scientific Computing Research, combines the fields of applied mathematics, scientific computing and machine learning to make stronger predictions about decomposing nuclear waste and other poorly understood systems.
“Physical models of many complex natural and engineering systems are, at best, partially known.” Perdikaris says. “Computational tools based on first physical principles can help us simulate, analyze and optimize systems ranging from blood cells to airplane wings. However, there are many cases for which traditional approaches to modeling and simulation face big challenges, either due to lack of modeling knowledge, or uncertainties associated with the problem setup.”
“Now that we have access to powerful computing abilities,” he says, “we can use data to make predictions about the physical world when mathematical models are not enough. What I’m trying to do is develop new mathematical methods and data-driven algorithms for analyzing and optimizing complex engineering systems.”
One of those systems is the Hanford site, a decommissioned nuclear production complex in Washington state, which left behind 56 million gallons of nuclear waste in leaking storage tanks when operations ended in 1989. Perdikaris and his research team will spend the next five years developing algorithms that could help predict the waste’s penetration underground, making them key players in the DOE’s excruciatingly slow battle to stop the leak before the nuclear waste hits the water table and contaminates even more groundwater.
“We would like to gain a predictive understanding of subsurface transport at the Department of Energy’s Hanford site,” Perdikaris said, “but even when sufficiently accurate mathematical models are available, the constitutive laws that characterize the subsurface material properties are entirely unknown. To compensate for this lack of knowledge we aim to develop a hybrid machine learning framework that can seamlessly synthesize models, governing equations and data.”
Perdikaris hopes his approach will create a new interface between the worlds of applied mathematics and machine learning.
“My approach aims to address fundamental challenges in each of these fields,” Perdikaris said. “But it is also trying to reveal an intricate synergy between them, too.”
Perdikaris will receive $150,000 per year over the course of five years, which will support two Ph.D. students and one postdoctoral research assistant in his lab. They will work closely with the DOE’s Pacific Northwest National Laboratory and Hanford Site.
The Office of Science of the Department of Energy selected 84 scientists from across the nation to receive funding as part of the 2018 Early Career Research Program, including 54 from U.S. universities. The program, now in its ninth year, supports the development of individual research programs of outstanding scientists early in their careers and stimulates research careers in the disciplines supported by the DOE Office of Science.