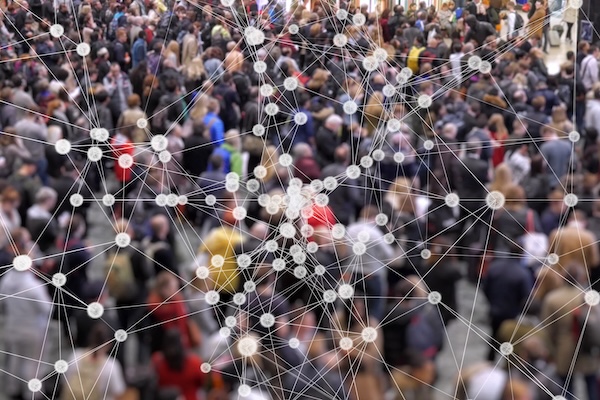
Epidemic modeling is a framework for evaluating the location and timing of disease transmission events, and is a part of the larger field of human mobility science. The COVID-19 pandemic put existing epidemic models to the test, with many institutions and corporations employing models that utilized smartphone location data to measure human-to-human interactions and better understand potential transmissions and social distancing.
Global Positioning System (GPS) data, aggregated into massive human mobility datasets —nmined from thousands of smartphone apps in which users opt-in to location sharing — has an unprecedented precision that can revolutionize human mobility science, epidemiology in particular, and brings the promise of monitoring potential disease transmission events — in real time — during epidemics like COVID-19. Subsequently, researchers can determine if two people are at the same location at the same time and assess whether any two people in the same city have the chance to encounter one another and spread an infection.
Because this data is commercial, researchers interested in building epidemic models based on this data face limitations related to data privacy, quality and multiple methodological choices that might bias any findings. In a recent paper in Nature Computational Science, CSSLab Post-Doctoral Researcher Francisco (Paco) Barreras and CSSLab Director and Founder Duncan J. Watts discuss the nature and implications of these challenges. They determined that to improve the usefulness of this class of data, it is necessary to identify best practices from existing studies on human mobility, identify “sharp edges” in the data processing, and increase transparency and accessibility so that findings with important policy implications will be more robust.