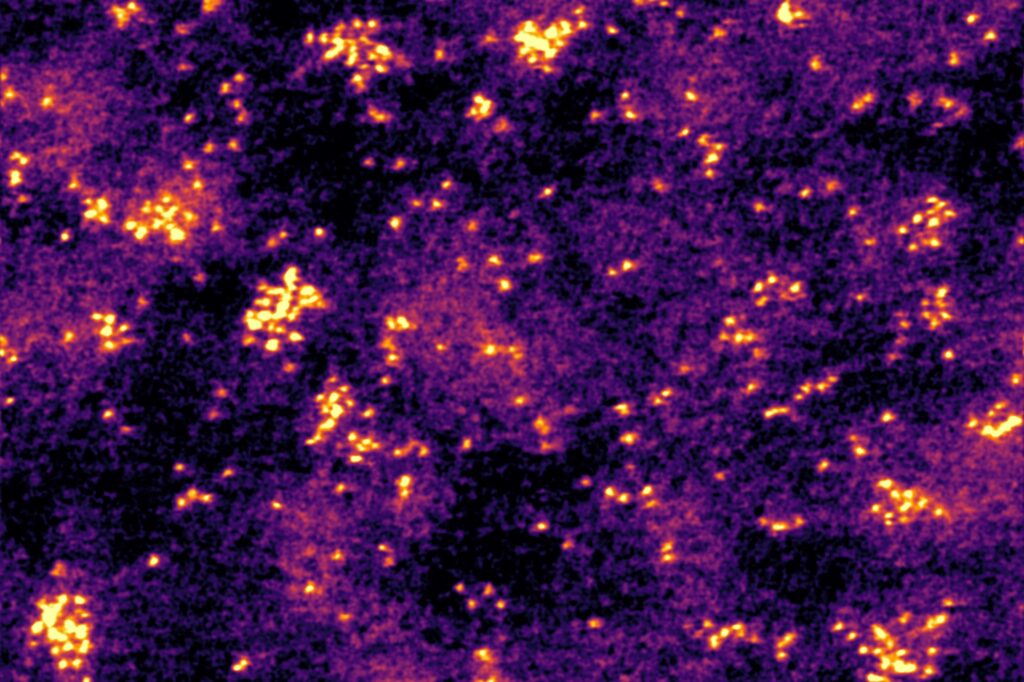
Imagine standing in a desert under a clear, starlit sky,” says Eric Stach, Robert D. Bent Professor of Engineering at the University of Pennsylvania. “With just your naked eye, you might spot the shimmering band of the Milky Way or the fuzzy glow of Andromeda. But without a telescope and other sophisticated tools, it’s nearly impossible to distinguish individual stars or truly understand their arrangement in the cosmos.”
Stach likens this experience to the challenge the team faced in trying to visualize molecular catalysts, the microscopic structures key to chemical reactions like converting carbon dioxide (CO₂) into usable fuels, on surfaces of semiconductor materials.
These catalysts, which contain heavy metal atoms, are scattered across surfaces in ways that are crucial to their performance, yet, like stars in the night sky, “their precise placement and clustering are difficult to discern with conventional techniques,” Stach says.
To that end, Stach and his collaborators at the University of North Carolina at Chapel Hill (UNC) and Yale University—working together as part of the Center for Hybrid Approaches in Solar Energy to Liquid Fuels— combined atomic-resolution imaging with machine learning analysis to better characterize the distribution of molecular catalysts. The team published their findings on the determination of the conditions, behaviors, and qualities of different catalysts in the journal Matter.
“The project brought together researchers with complementary expertise in imaging, molecular synthesis, catalysis, and surface chemistry,” says Jillian L. Dempsey of UNC. “The collaboration was essential for visualizing how individual catalysts are distributed across semiconductor photoelectrodes.”
By providing a new understanding of how molecular catalysts behave on semiconductor surfaces, the team’s findings pave the way for more efficient catalytic systems. Advances could accelerate developments in renewable energy technologies, such as CO₂ conversion and hydrogen production, and offer insights applicable to a wide range of industrial processes.
“The elegance of our approach really lies in a simple yet powerful idea,” says Sungho Jeon, a postdoctoral researcher in the Stach Group and co-first author of the paper. “If you want to correlate variables, like how molecular coverage and distribution influence catalyst performance, you first have to measure them accurately. Our work shows how to precisely and robustly measure surface coverage, quantify distributions, and see how changing conditions, like the type of molecule or functionalization process, alters those properties.”
This story was written by Nathi Magubane. To read the full article, please visit Penn Today.