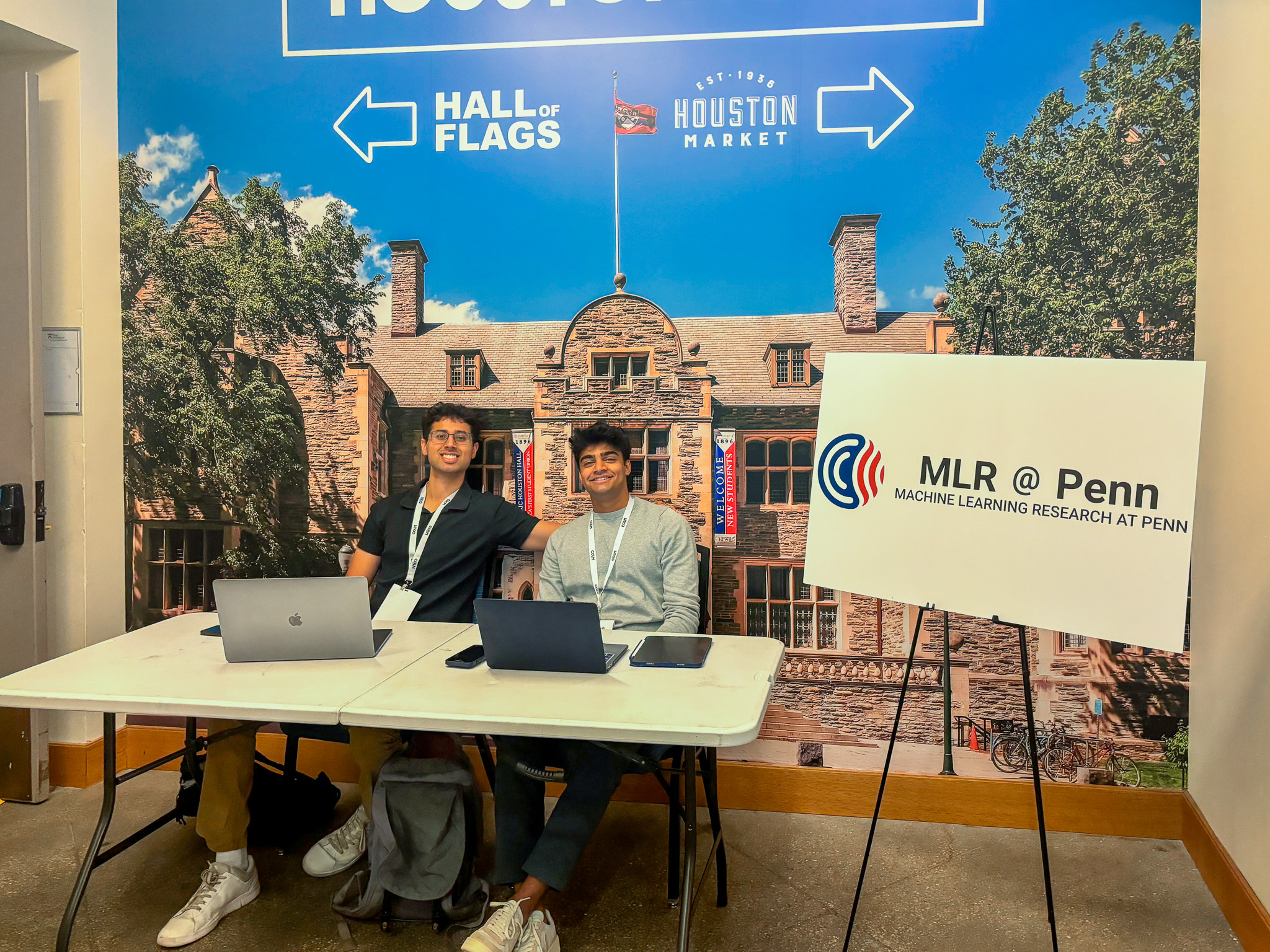
Last year, researchers published over 50,000 papers on machine learning — a nearly 50% jump from the previous year. “Figuring out what’s worth reading is hard,” says Alok Shah (CIS’26, ESE’26, C’26), president of Machine Learning Research @ Penn (MLR@Penn), a club that helps students stay on top of fast-moving developments in AI.
Reading academic papers, especially about cutting-edge research, can be a tricky endeavor. Every advance in AI builds on prior work, so understanding one paper might require diving into the citations to find (and then read) many more.
“Quickly judging a paper’s technical merit and broader impact? That’s not something I learned in class,” Shah adds. “It’s a skill I’ve been trying to build — and honestly, I’m still working on it.”
Supporting Student AI Research
Recognizing this gap is what led to the creation of MLR@Penn. “Our club provides an avenue for students to interface with and contribute to research at the frontier of AI,” says Keshav Ramji (CIS’24, GEng’24, W’24), who founded the club in 2023, and now works at IBM Research AI.
The group quickly grew from a small core to dozens of students, majoring in everything from computer science to bioengineering. “Machine learning is an interdisciplinary field that spans all of the undergraduate schools at Penn,” notes Shah.
Ramji founded the student organization after reflecting on how much he would have benefitted from a peer-led introduction to the world of academic research. “I reached this point in junior year,” he recalls, “when I could read papers and come up with new ideas — that’s when I realized I wanted to share this experience with other undergrads, especially first-years and sophomores, who may be trying to break into the field.”
Reading Group
One of the main ways MLR@Penn prepares undergraduates for research is by discussing academic papers in small groups, much like book clubs would dissect a novel. “By putting our heads together,” says Alexander Kyimpopkin (EE’25, GEng’25), a member of the club, “we’re able to understand papers better and keep up with the state of the field.”
This notion of a “reading group” is commonplace in the lives of graduate students and industry researchers but is rarely accessible to undergraduates outside of on- or off-campus internships. “It’s an effective forum to talk about the latest cutting-edge research, like you would in industry or academia, but tailored for students with less experience,” says Ramji.
Two years ago, Ramji and Kyimpopkin traveled to New Orleans for the annual Conference on Neural Information Processing Systems (NeurIPS) to present a new approach for analyzing decision making in large language models (LLMs).
Last December, Ramji and Shah also traveled to Vancouver and presented two novel methods for improving LLM reasoning at NeurIPS. “Our experience shows it’s possible for undergraduates to come up with high-quality ideas and work towards publishing them,” says Ramji.
Solving Real-World Problems
In addition to the reading group, MLR@Penn also regularly hosts industry speakers to help students understand what career opportunities exist in AI. “The linkage between academia and industry is important,” says Shah. As the club’s leaders point out, that link runs in two directions: it’s not only helpful for students to learn how industry is using academic research, but also critical for business leaders to keep abreast of AI research.
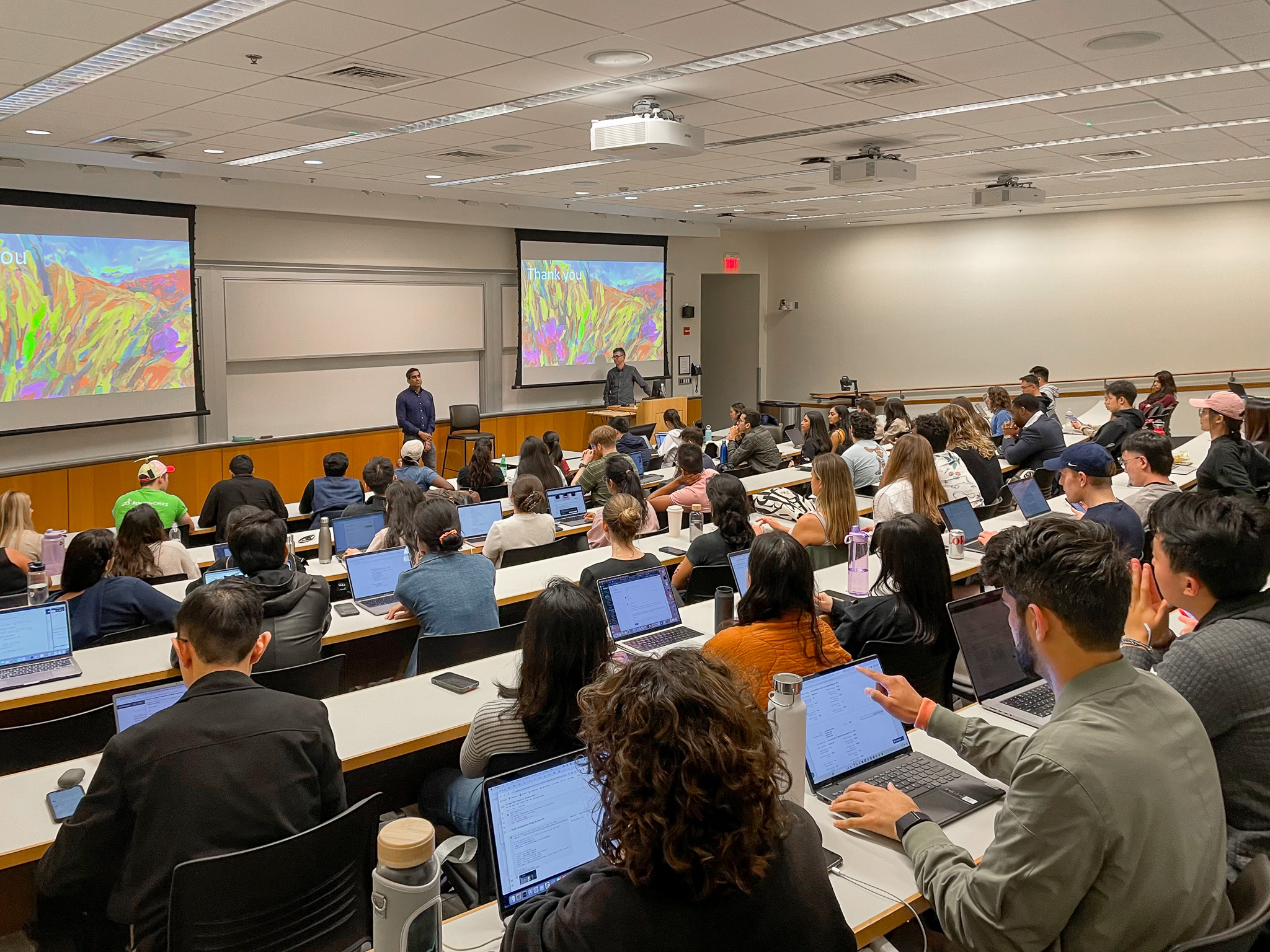
So far, the group has hosted speakers like OpenAI’s Amol Shah (W’06), Stefano Puntoni, Co-director of AI at Wharton, and Bryan Kim of Andreesen Horowitz, the well-known venture capital firm in Silicon Valley. Last fall, the club put together a major workshop alongside the Conference on Language Modeling hosted at Penn, inviting more than a dozen speakers from universities across the United States along with firms like Google, Apple and IBM.
Ultimately, MLR@Penn aims to equip members with the tools to solve real-world challenges. Last year, Ramji contributed to Granite 3.0, IBM’s family of open-source language models, and other alumni have gone on to leverage their AI training in robotics and finance.
“ChatGPT made the broader public aware of AI’s potential,” says Ramji. “But there are still plenty of open questions in language modeling — especially in balancing the development of performant and capable models with trustworthiness and safety — where there’s a lot more progress to be made.”
Students interested in joining MLR@Penn can visit the club’s webpage. Alumni and industry partners interested in collaborating with the club should reach out to pennmlresearch@gmail.com. Interested Penn undergraduates can register for the Penn AI Hackathon on April 18-19, 2025.