Translating the World’s Languages
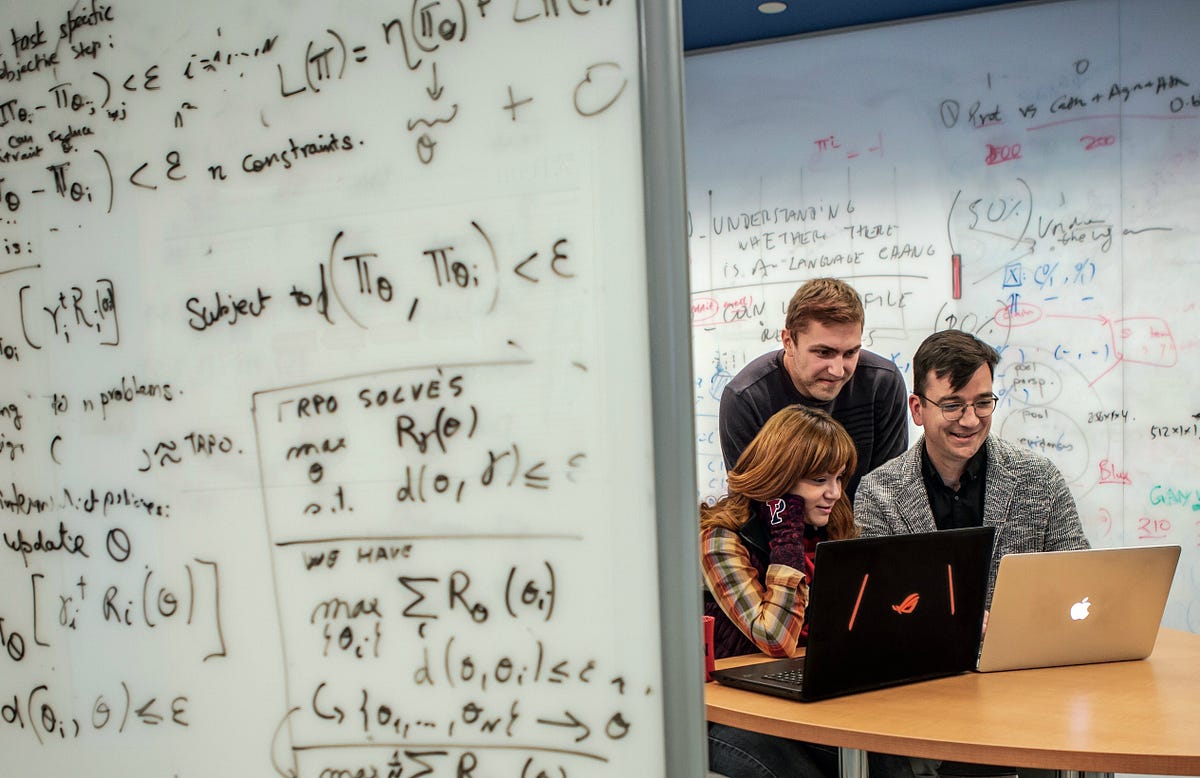
By Jessica Stein Diamond
Chris Callison-Burch first wondered how computers could be used to translate languages as a high school freshman while tinkering with his first computer and reading science fiction. Classics such as The Hitchhiker’s Guide to the Galaxy featured creative linguistic plot devices like “Babel fish,” which when stuffed in an ear allowed instant comprehension of any language.
“Opening up communication between all people is still really my goal,” says Callison-Burch, laughing at the improbable scope of his now decades-long ambition, which he’s uniquely equipped to pursue.
OVERCOMING OBSTACLES
Known for his knack for addressing bottlenecks and obstacles to advance computerized translation performance, Callison-Burch works at the intersection of linguistics and the computer science field of machine learning, using artificial intelligence to automatically learn from experience. An associate professor in Computer and Information Science, Callison-Burch similarly strives to lower barriers to entry for students interested in computer science and to research topics that benefit human welfare and dignity.
“What drew me to the field of computational linguistics was that if you could make this technology work, then the value in terms of society, economics, culture and understanding would be huge,” he says, noting real-time translation’s value for global security, commerce and disaster recovery. For example, many people affected by Haiti’s 2010 earthquake texted requests for help in Haitian Creole, yet because so few first responders knew the language, critically needed assistance was delayed and thousands of people ultimately died.
“My research is guided by trying to address languages that haven’t yet made it into Google Translate,” Callison-Burch says. His research group has developed novel cost- and time-saving methods to translate languages using crowdsourcing and images, breakthroughs first published in 2011 and 2018, respectively. These methods offer great promise for generating translations beyond the current set of 100 languages on Google Translate, potentially expanding to even more of the world’s known 7,000-plus languages.
“Chris is a top influencer in our field who has changed how we do things for machine translation research,” says Kevin Duh, senior research scientist at the Johns Hopkins University Human Language Technology Center of Excellence. “Whenever we need to build a new machine translation system, we follow his procedure of collecting data from the Amazon Mechanical Turk, hiring workers via this global marketplace for crowdsourced labor. His use of the wisdom of the crowd as applied to translation gives us a way to get better results quicker with fewer resources.”
ENGINEERED DICTIONARIES
In 2018, Callison-Burch’s research group shared another promising new translation method for some of the world’s most difficult-to-translate languages. They used images (for instance, of a cat) plus vast quantities of crowdsourced data identifying linked words for each image to create reverse-engineered dictionaries for 10,000 words in 100 languages. “We’re building on an insight that images are somehow interlingual, that an image of a cat is the same whether you speak English or Indonesian, so we can use simplified representations of images to train the model,” says Callison-Burch. “This language-independent way of thinking about words through their visual representations allows us to use a new type of data to learn translations.”
Even though Google Translate is as close as possible to the state of the art for 100 languages, the quality is wildly different between languages like French and Arabic, and languages like Indonesian and Urdu. Callison-Burch’s “Massively Multilingual Image Dataset,” published in the Proceedings of the Association for Computational Linguistics (ACL), addresses crucial gaps in machine learning translation techniques. Prior methods required costly data from professional translators and vast quantities of online texts such as websites, books and newspapers. This approach worked for the 24 “high-resource” languages of the European Union but wasn’t useful for “low-resource” languages with fewer speakers, sparse or nonexistent translation budgets, and scarce language texts online.
Daphne Ippolito, doctoral student and ACL paper first co-author, describes the image data set “as an interesting and necessary step that allows us to stop relying on experts for translation and to try to gather our data from whomever and wherever we can. The paper further shows that our algorithms tend to work better for translating words that are more concrete such as ‘house’ or ‘sailing’ regardless of their part of speech.”
John Hewitt (CIS’18), also first co-author, says, “We released the data and code because the paper provides proof of concept that methods using visual information can be useful for low-resource languages. It provides the first data set for researchers to explore the utility of visual symbol representation at a large scale, allowing others to improve upon the methods we’ve developed.”
Derry Wijaya, who grew up in Malang, Indonesia, and is now an assistant professor at Boston University, joined Callison-Burch’s group as a postdoc in 2016. “My Ph.D. advisor gave me a list of people who were doing out-of-the-box research that is fun, exciting and at the same time has big impact for natural language processing,” says Wijaya. “Chris was first on that list. He taught me how to navigate my first academic career negotiation and placement.” She appreciates how Callison-Burch helped her develop skills in advising students and writing grants, and how he guided her and other early-career faculty as they submitted a grant that helped them develop visibility with a key funder, the Defense Advanced Research Project Agency (DARPA).
“Chris also helped me to adjust my communication style. I grew up in Javanese culture, which has a concept of self-restraint,” says Wijaya. “I’m now more direct in discussions about research, especially in giving others feedback. This helps me have more confidence, which is good.”
BEYOND EXPECTATIONS
Mindful of how his own undergraduate experiences launched his career, Callison-Burch encourages undergraduates to contribute to his research group. “Being open to anyone who wants to try out research has paid dividends that are wildly beyond my expectations,” says Callison-Burch. In 2017–2018, 40 undergraduates worked in his lab alongside eight graduate students and two postdocs. This fall he began teaching an AI course to a cohort of 100 students; 250 students were wait-listed, and he plans to expand the enrollment cap in the future.
Broader concerns inform his research as well. To address the risk that low-wage workers associated with Amazon Mechanical Turk might experience “digital sweatshop” conditions, Callison-Burch developed a Chrome plugin extension called Crowd Workers to help participants find higher-paying work. His group has a pending grant to explore ways to help people living in rural U.S. communities to develop skills to earn a living wage via Amazon Mechanical Turk.
Amid these broad goals Callison-Burch retains a humble perspective, ruefully admitting, “I’m embarrassingly monolingual for someone who works on translation. The machine has me beat; it’s much more capable of learning languages than I am.”