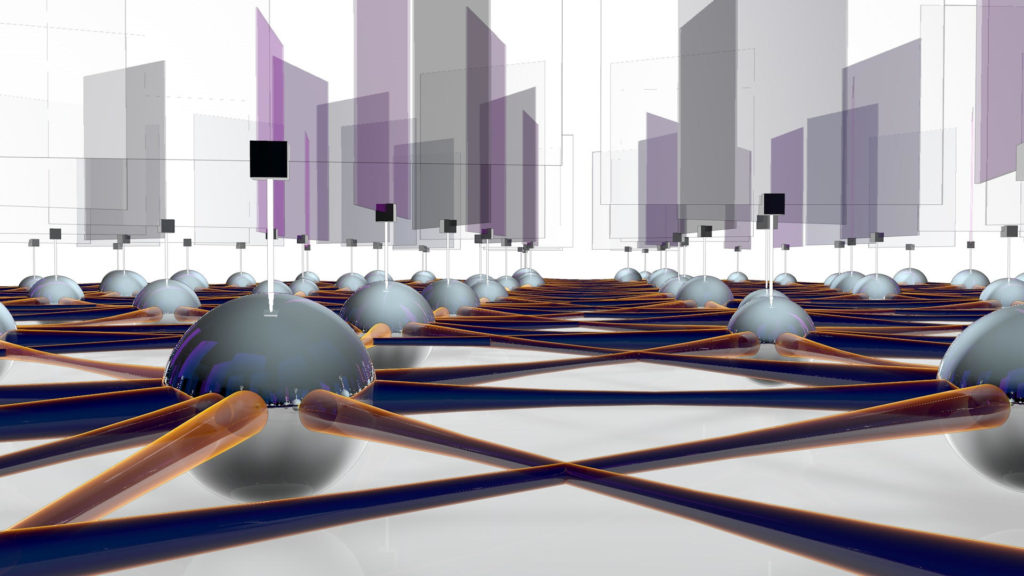
Research published in the SIAM Journal on Applied Mathematics describes a new mathematical model for studying influence across social networks. Using tools from the field of topology, Robert Ghrist and Ph.D. graduate Jakob Hansen developed a framework to track how opinions change over time in a wide range of scenarios, including ones where individuals can use deceptive behaviors and propaganda agents can drive a group’s consensus.

With the rise of social media platforms, there has been increased interest in developing different types of models to study behavior over networks; in mathematics, that means studying networks, groups of individuals, known as nodes, and their connections to one another, known as edges. The current challenge, says Ghrist, is developing mathematical frameworks that can incorporate a broader range of features to help model more real-world types of scenarios.
“There are a lot of people putting out models that have one or or two novel features; one allows for multiple opinions, another allows people to selectively lie to their neighbors, and another has the introduction of a propagandist,” he says. “What we were looking to do was come up with a framework that can incorporate all of these different aspects, yet still be able to prove rigorous theorems about how the model behaves.”
To do this, Ghrist and Hansen used topological tools called sheaves, previously used in their group. Sheaves are algebraic data structures, or collections of vector spaces, that are tethered to a network and link information to individual nodes or edges. Using a transportation network as an illustrative example, where train stations are nodes and the tracks are the edges, sheaves are used to carry information about the network, such as passenger counts or the number of on-time departures, not only for specific stations but also on the connections between stations.
“These vector spaces can have different features and dimensions, and they can encode different quantities and types of information,” says Ghrist. “So the sheave consists of collections of vectors over top of each node and each edge with matrices that connect them all together. Collectively, this is a big data structure floating over top of your network.”
Continue reading at Penn Today.