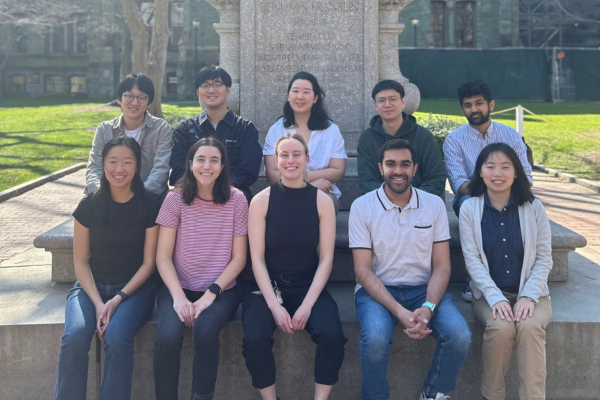
AI tools are being rapidly integrated into our daily lives, therefore their responsible development and regulation are paramount. This is the core mission of Penn Engineering’s ASSET (AI-Enabled Systems: Safe, Explainable and Trustworthy) Center.
To support that mission and the next generation of engineers actualizing it, Amazon Web Services (AWS) has granted Penn Engineering $700,000 to fund 10 Ph.D. student research projects in advancing safe and responsible AI. Eleven of Penn’s world-class faculty members working in the ASSET Center, part of Penn Engineering’s Innovation in Data Engineering and Science (IDEAS) Initiative, are advising the students in their work.
“With the current proliferation of media stories about vulnerabilities and the potential harm of AI, it is critical to bridge the trust gap between developers of AI technology and skeptical users now so we can realize the promise of AI,” says Rajeev Alur, Zisman Family Professor in Computer and Information Science (CIS) and Director of ASSET. “The generous gift by AWS in support of ASSET’s mission to develop the foundations necessary for making AI-based systems trustworthy can help bridge this gap. We are extremely grateful for their support.”
Amazon Web Services has given these funds with the sole intention of supporting research that advances responsible AI innovations.
“We are delighted to continue the collaboration with Penn that now spans across safety, interpretability, fairness, as well as natural language processing, reinforcement learning and beyond,” says Stefano Soatto, Vice President of Applied Science for Amazon Web Services AI. “It is a momentous time in AI, and both academia and the technology industry stand to benefit from the collaboration. Being at the forefront of AI services, AWS provides a window into challenging and often unexplored research problems that can inspire the creative endeavors of students and faculty, who then contribute to the open research community advancing the state of knowledge for the benefit of all.”
The 10 Ph.D. students were identified by Penn Engineering faculty and selected as recipients by the ASSET Center committee with input from the AWS team. With responsible AI at their core, these projects span across large language models (LLMs), deep learning and human-algorithm interactions, all of which are fundamental areas of AI research that require innovative approaches. Below is the list of students and summaries of their research projects.
Alaia Solko-Breslin is advised by Rajeev Alur. Solko-Breslin’s research is focused on developing neurosymbolic learning algorithms that are compatible with black-box programs. She is exploring techniques to facilitate learning when programs cannot be differentiated explicitly.
Young-Min “Jeffrey” Cho is advised by Lyle Ungar, Professor in CIS, and Sharath Chandra Guntuku, Research Assistant Professor in CIS. Cho studies LLM-based conversational agents, exploring how to generate strong clarifying questions, control bot response length and support multi-party conversations. He is also working on deriving social and psychological insights from language-based assessments, such as mental health chatbots and cultural differences in emotional expressions.
Ira Globus-Harris is advised by Michael Kearns, National Center Professor of Management & Technology in CIS and an Amazon Scholar, and Aaron Roth, Henry Salvatori Professor of Computer & Cognitive Science in CIS, who is also an Amazon Scholar. Globus-Harris’ work focuses on algorithmic fairness for machine learning, specifically in developing flexible algorithmic techniques which align fairness and model accuracy.
Kyurae Kim is advised by Jacob Gardner, Assistant Professor in CIS. Kim’s work is on developing and evaluating statistical inference algorithms. In particular, she works on variational inference, stochastic gradient Markov chain Monte Carlo. Her work aims to theoretically analyze these algorithms with a particular focus on analyzing their real-world behavior to guide their development for practical applications.
Jiahui Lei is advised by Kostas Daniilidis, Ruth Yalom Stone Professor in CIS. Lei’s research focuses on the algorithms and representations for 3D and 4D geometric data, including the representation, reconstruction and generation of 3D and 4D dynamic objects and scenes.
Rahul Ramesh is advised by Pratik Chaudhari, Assistant Professor in Electrical and Systems Engineering (ESE) and in CIS. Ramesh is interested in understanding the role of data in deep learning. His work seeks to explain why large amounts of data can be used to train models to tackle many different tasks and identify how such models could fail by characterizing the geometry of the space of tasks.
Mirah Shi is also advised by Kearns and Roth. Her work focuses on algorithms for learning in sequential, game-theoretic settings. She is currently studying how to make predictions that are reliable and adaptable for broad, downstream decision-making tasks.
Darshan Thaker is advised by René Vidal, Rachleff University Professor in ESE and Radiology with a secondary appointment in CIS. Thaker’s work is focused on developing principal machine learning methods that are robust to input corruptions, such as malicious or adversarial inputs or natural image artifacts.
Stephanie Wang is advised by Danaë Metaxa, Raj and Neera Singh Term Assistant Professor in CIS and in the Annenberg School for Communication. Wang studies human-algorithm interaction in social computing applications. Her latest work expands upon methods in socio-technical auditing to examine the impact of social-media news feed algorithms on news curation and user behaviors and perceptions.
Weiqiu You is advised by Eric Wong, Assistant Professor in CIS. You’s research is focused on faithful-by-construction explainable machine learning (ML) models with groups of features as explanations. Her research spans from vision to language and applies to scientific discovery in cosmology and trustworthy ML in surgical settings. Her goal is to develop structured explanations that are faithful to the models and useful to the users.